Announcing the 2023 CCAI Innovation Grants Awardees
9 proposals have been awarded a total of $1.4M USD as part of the second CCAI Innovation Grants program
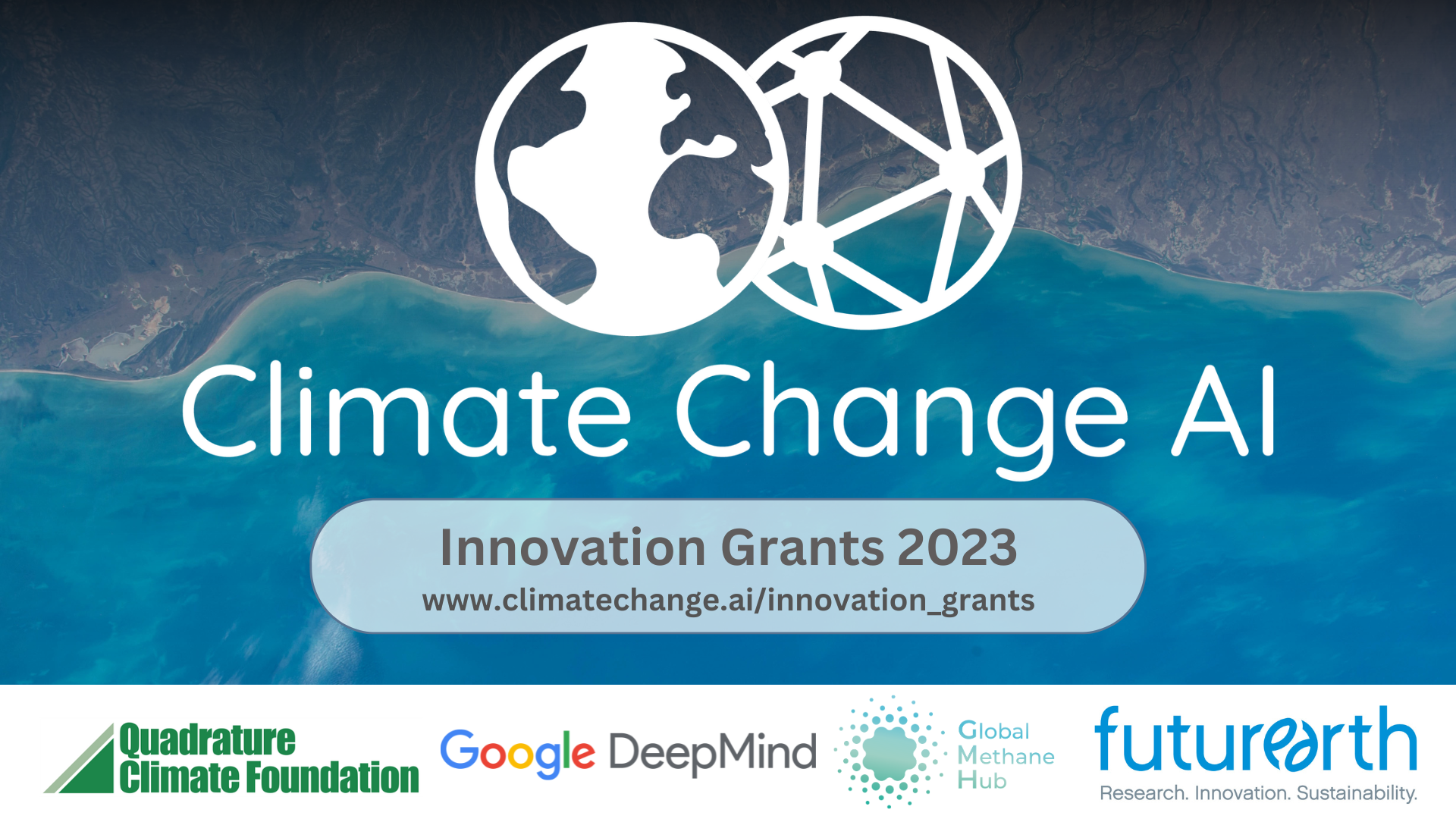
Climate Change AI is excited to announce the awardees of our 2023 Innovation Grants Program. This program, now in its second cycle, supports impactful projects leveraging AI and machine learning to address problems in climate change mitigation, adaptation, and climate science, as well as the creation of publicly-available datasets/simulators to catalyze further work in this area.
To select our 2023 cohort, submissions from 47 countries were peer-reviewed by an international committee of experts in AI and climate change-relevant fields. The awardees include nine outstanding projects spanning 30 universities, companies, nonprofits, NGOs, and governmental organizations across nine countries and five continents, bringing together interdisciplinary teams for projects touching many aspects of the climate crisis. A key aspect of all the projects is a well-developed pathway to climate impact, generally instantiated through strong partnerships with entities key to deployment.
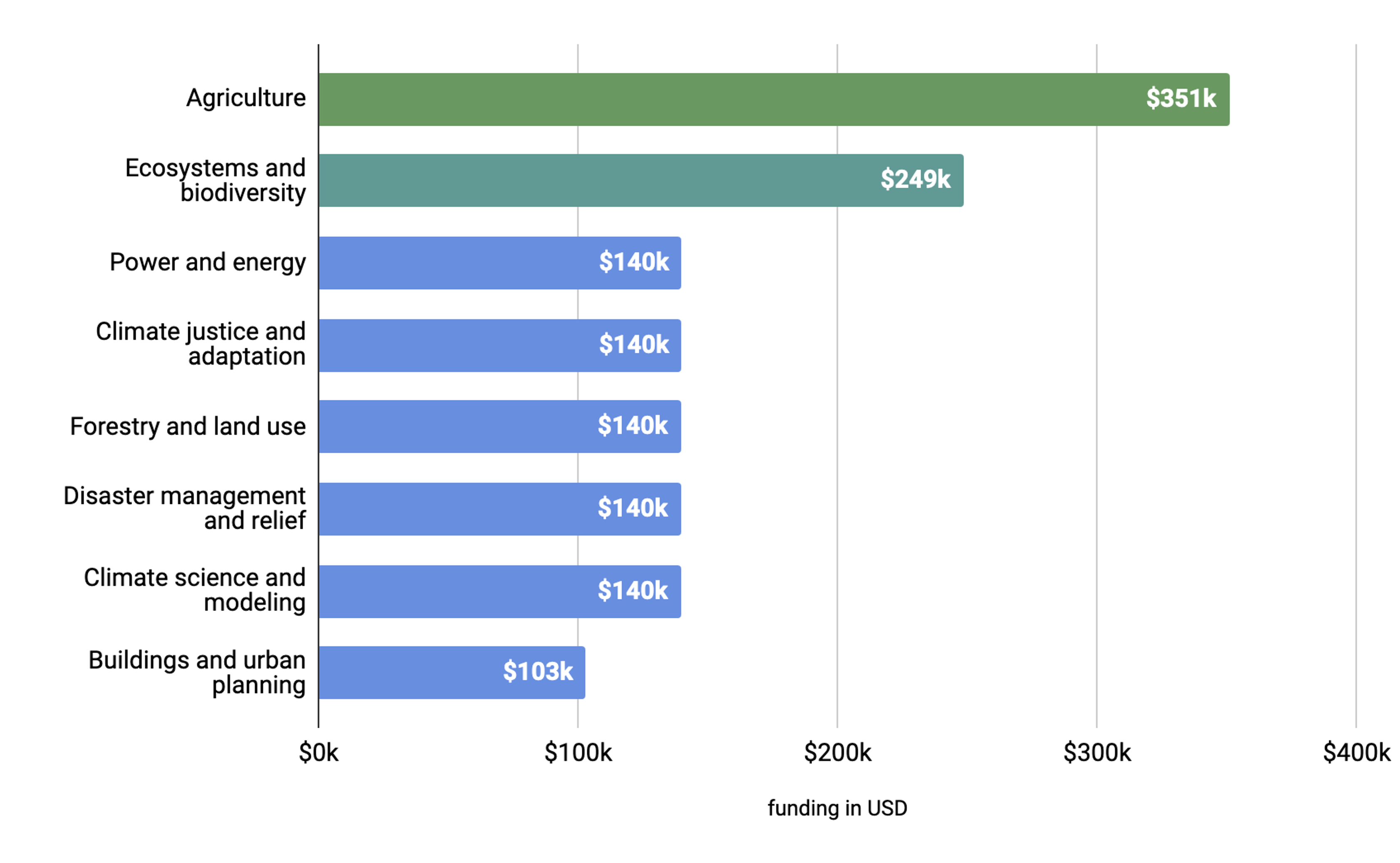
The funded proposals are listed below (in alphabetical order), and more information is available at www.climatechange.ai/innovation_grants.
![]() |
Artificial intelligence for water management in the Red River Delta to meet the water demand and control saline intrusion in a changing climate Ivan Serina, Roberto Ranzi (Università di Brescia), Ngo Le An (Dai hoc Thuy Loi), Toan Trinh (University of California Davis) This project utilizes AI techniques to optimize water management in the Red River Delta in Vietnam, where a complex network of dams serves multiple purposes, including hydropower generation, flood regulation, agriculture water supply, and controlling seawater intrusion. The AI algorithms will offer optimal management policies for reservoir operations, focusing on water supply for agriculture and energy production during low-flow seasons while addressing constraints and climate conditions |
![]() |
Curbing Illegal Logging Patterns using Sound-Based Detection Techniques Clara Zemp (Université de Neuchâtel), Henry Muchiri (Strathmore University), Anthony Mwangi (Kenya Forestry Research Institute), Fengshou Gu (University of Huddersfield) Illegal logging in Kenyan community forests is contributing to increased carbon emissions and negatively impacting the livelihoods of local communities. This project aims to use IoT devices to detect logging sounds, sending alerts for further investigation, and conducting carbon stock measurements to assess the impact, promoting data-driven forest management for climate change mitigation and benefits to local communities. |
![]() |
Data Extraction and Modelling from Plant Trait Literature Richard Reeve, Christina Cobbold (University of Glasgow), Neil Brummitt, Ana Claudia Araujo, Ben Scott (London Natural History Museum), Claire Harris, Glenn Marion (Biomathematics and Statistics Scotland) The project aims to create a comprehensive global database of plant traits related to climate and habitat, utilizing computer vision and natural language processing techniques to process data from natural history collections and ecology literate. This database will provide essential information to improve global biodiversity-climate models, in turn contributing to policy efforts to preserve biodiversity in the face of changing climatic conditions. |
![]() |
Developing machine learning tools to rapidly assess the catastrophic impact of a range-extending sea urchin in a global warming hotspot Arie Spyksma, Kelsey Miller, Katerina Taskova (University of Auckland), John Keane, Nicholas Perkins (University of Tasmania), Ariell Friedman (Greybits Engineering) In Australia and New Zealand, growing climate-induced sea urchin population growth poses a significant threat to kelp-dominated reefs. To address the threat, this project aims to develop machine learning to rapidly analyze underwater imagery to assess sea urchin expansion and kelp decline, providing essential data for proactive ecosystem management. |
![]() |
EMPIRIC_AI: AI-enabled ensemble projections of cyclone risk for health infrastructure in Pacific Island Countries and Territories Christopher Horvat, Michelle McCrystall (University of Auckland), Berlin Kafoa (The Pacific Community), Elizabeth McLeod (World Health Organization), Craig McClain (Harvard Medical School) Communities in Pacific Island Countries and Territories (PICTs) face significant climate change impacts and their healthcare infrastructure is expected to be under extreme pressure in the coming decades. This project will develop high resolution climate models and targeted cyclone risk projections for health infrastructure in PICTs. This work will provide decision-makers and the public access to new datasets and tools with dedicated, high-resolution projections of future risks and planning to support health resilience for frontline communities. |
![]() |
Flood Justice and Adaptation in the Rio Grande Valley of Texas with AI and satellite imagery Beth Tellman, Lucas Belury, Zhijie Zhang (University of Arizona), Ana Laurel (Texas RioGrande Legal Aid) Climate change is expected to increase flood risk in the Rio Grande Valley of Texas, as well as exacerbating inequities in exposure to floods, which disproportionately impact communities of color. However, inadequate flood risk data hampers adaptation efforts. This project plans to use machine learning and satellite imagery to create high-quality flood maps and a flood database for the Rio Grande Valley in order to support flood justice lawsuits and promote equitable flood adaptation. |
![]() |
From Observing Power to Improving Power: Loss Localization in the Distribution Grid through Topology June Lukuyu (University of Washington), Genevieve Flaspohler, Mohini Bariya, Joshua Adkins, Kwame Abrokwah, Noah Klugman (nLine Inc.) Meeting the energy demands of low- and middle-income countries (LMICs) while addressing climate change requires more efficient electric grids. The project, based in Ghana, aims at a cost-effective solution involving voltage sensing at customer connections and machine learning algorithms to detect and localize distribution grid losses, particularly in the low-voltage networks where most issues occur. |
![]() |
Mapping Rice Water Management and Methane Emissions in Ghana Sherrie Wang (MIT), Soren Vines, Freddie Monk (Aya Data), Benjamin Adevu, William Hunt (Demeter Ghana) Rice cultivation, while essential for agriculture, comes with methane emissions that are often poorly quantified in global climate analyses. This project aims to better understand and quantify methane emissions from rice cultivation, particularly in Ghana, by using satellite imagery and machine learning alongside field surveys to map rice flooding, a key factor influencing methane generation. |
![]() |
The CityLearn Challenge 2023 Zoltan Nagy, Javad Mohammadi (UT Austin) Reducing energy consumption and emissions from buildings is crucial, given that buildings contribute to 30% of greenhouse gas emissions. This project develops a representative model of energy use in neighborhoods, and executes a novel edition of the CityLearn Challenge, which tests the ability of advanced control agents for demand response and reduction of carbon emissions at the neighborhood level. The model aims to reduce energy use and / or shift operations to low emissions electricity. |
The icons used in the table above are obtained via remixicon.com.
We would like to thank Quadrature Climate Foundation, Google DeepMind, and Global Methane Hub for their financial sponsorship of the 2023 Innovation Grants Program. We are also grateful to the Canada Hub of Future Earth for serving as the fiscal sponsor.
For those interested in applying for our Innovation Grants program, the next cycle of the program is expected to open in the coming weeks.