From Observing Power to Improving Power: Loss Localization in the Distribution Grid through Topology
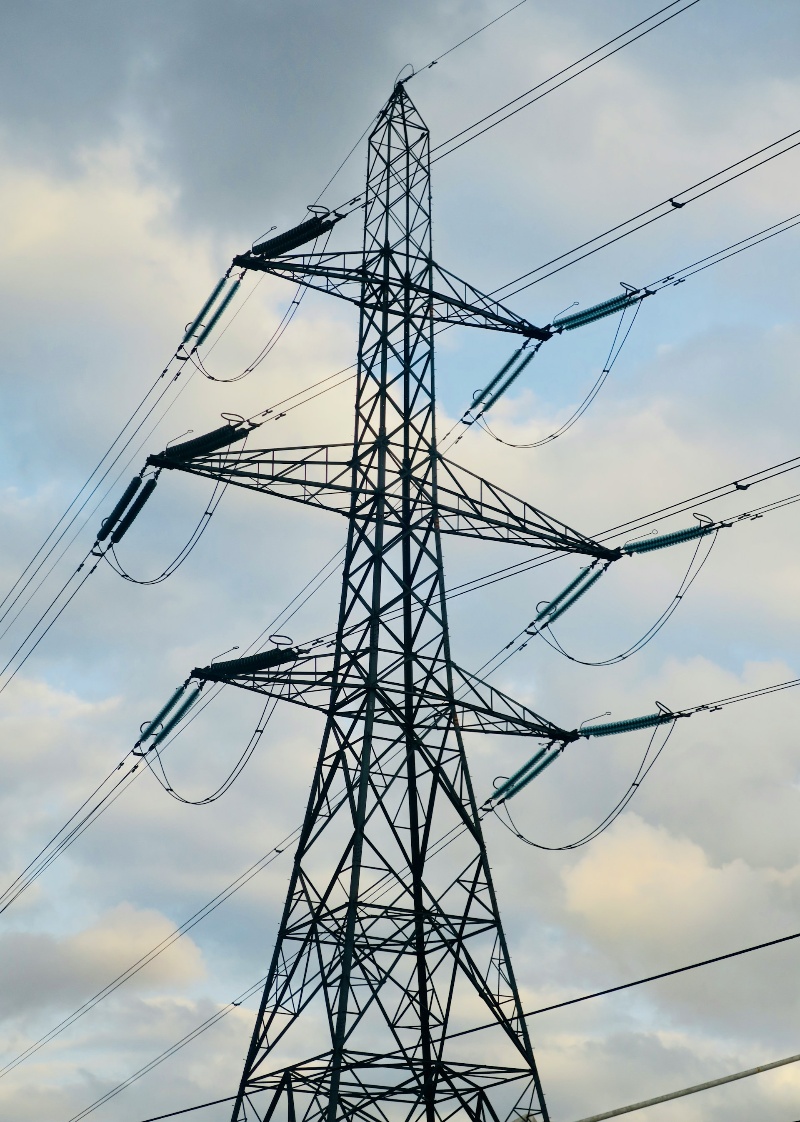
PI and co-PIs: June Lukuyu (University of Washington); Genevieve Flaspohler (nLine Inc.); Mohini S Bariya (nLine); Joshua Adkins (nLine Inc.); Kwame Abrokwah (nLine Inc.); Noah Klugman (nLine Inc.)
Funding amount: $140,000
Project overview: Meeting the energy demands of low- and middle-income countries while addressing climate change requires more efficient electric grids, reducing power loss from malfunctioning equipment and overloaded lines. This project, based in Ghana, aims at a cost-effective solution to this problem, involving machine learning algorithms to detect and localize distribution grid losses, particularly in the low-voltage networks where most issues occur. Identifying such issues promptly can better enable grid operators in Ghana and elsewhere to improve grid reliability and effectively meet power demand, lowering both costs and associated greenhouse gas emissions.
Full abstract:
Click to expand
Climate change mitigation and adaptation requires adequate, affordable, clean energy for all. Electric grids are central in this future and must shoulder new burdens, delivering energy from intermittent and distributed sources to meet growing demand. This challenge will be particularly acute for grids in low- and middle-income countries (LMICs), where per capita energy consumption is forecasted to grow dramatically but resources to develop and maintain grid infrastructure are limited. LMIC grids are already strained and weak, as evidenced by measures of network losses, which are often up to 30% in LMICs compared to a baseline of 5% in richer nations. Strengthening LMIC grids to meet climate challenges within resource constraints demands a new level of operational efficiency, particularly in the low-voltage distribution network where the majority of losses and issues originate. Detecting and targeting sources of loss and system weakness in the distribution grid, such as faulty transformers, overloaded lines, and malfunctioning regulating equipment, is critical for efficient grid operation. However, the distribution grid in LMICs is a vast, essentially un-sensed infrastructure; problems go long unnoticed and undiagnosed, leading to costly inefficiencies with human and environmental consequences. Existing approaches to detecting sources of loss in the distribution grid require sensing systems that would take decades to enable at scale and are prohibitively expensive in LMICs. We propose an alternative: a low-cost, flexible framework that couples voltage sensing at subsampled customer connections with machine learning algorithms to estimate grid topology and localize losses in distribution networks.
Power & Energy Societal Adaptation & Resilience Unsupervised & Semi-Supervised Learning