Curbing Illegal Logging Patterns using Sound-Based Detection Techniques
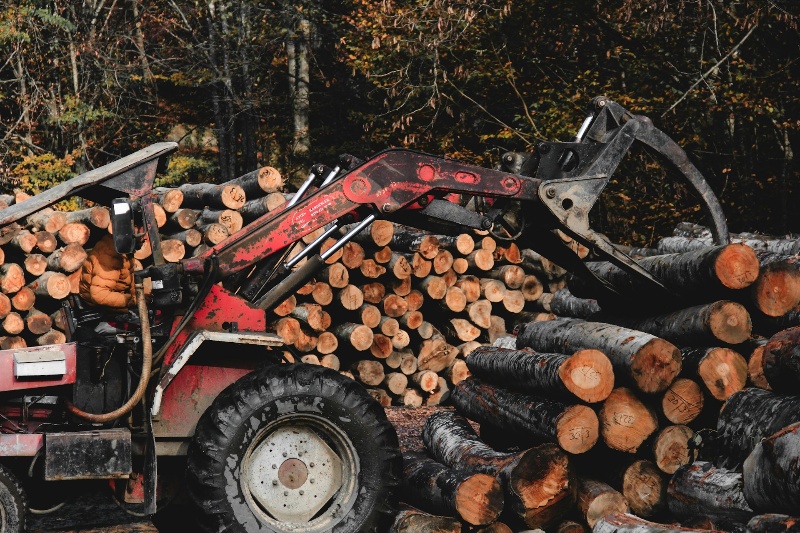
PI and co-PIs: Delphine Clara Zemp (Université de Neuchâtel, Labo biologie de la conservation); Henry Muchiri (Strathmore University); Anthony Mwangi (Kenya Forestry Research Institute); Andreas Jedlitschka (Fraunhofer IESE); Fengshou Gu (University of Huddersfield)
Funding amount: $139,500
Project overview: Illegal logging in Kenyan community forests is contributing to increased carbon emissions and negatively impacting the livelihoods of local communities. This project aims to use IoT devices to monitor forest audio, developing machine learning algorithms to classify sounds from chainsaws and axes, which are then flagged for intervention. By reducing the need for active monitoring, this initiative directly benefits community forest associations, and impact on study locations will be measured directly using carbon stock assessment against controls.
Full abstract:
Click to expand
Illegal logging plagues community forests in Kenya, affecting the amount of carbon sequestration and consequently increasing the rate of carbon emissions. Moreover, it affects the livelihoods of communities that directly depend on these forests, by straining resources for their household economies. The proposed solution involves deploying IoT devices that detect sound signals produced by chainsaw and axe, which are popular tools for logging. These signals are then transmitted over a wireless network to a cloud-based platform for storage and classification by a neural network architecture. In case of a positive result, a drone is sent to the site of illicit logging and images are captured for further analysis. The validity of this solution will be certified by measurement and analysis of carbon stocks before, during, and after the period of implementation. This guides in establishing the rate of illegal logging and its contribution to climate variability and change. This study aims to justify a data-driven approach in forest management, where results of data analyses guide decision making processes for climate mitigation and adaptation. The research proposes to publish datasets on environmental sounds, sounds produced by axe and chainsaw, and spatial carbon maps. The latter two datasets seek to address current research gaps in sound classification and carbon analysis respectively. Community forest associations within these forest-dependent communities are set to directly benefit, by relieving them from active monitoring for illegal logging activities. During deployment, women and youth-led groups will be prioritised to enhance inclusivity in forest management and climate action.
Carbon Capture & Sequestration Computer Vision & Remote Sensing Forests