Developing machine learning tools to rapidly assess the catastrophic impact of a range-extending sea urchin in a global warming hotspot
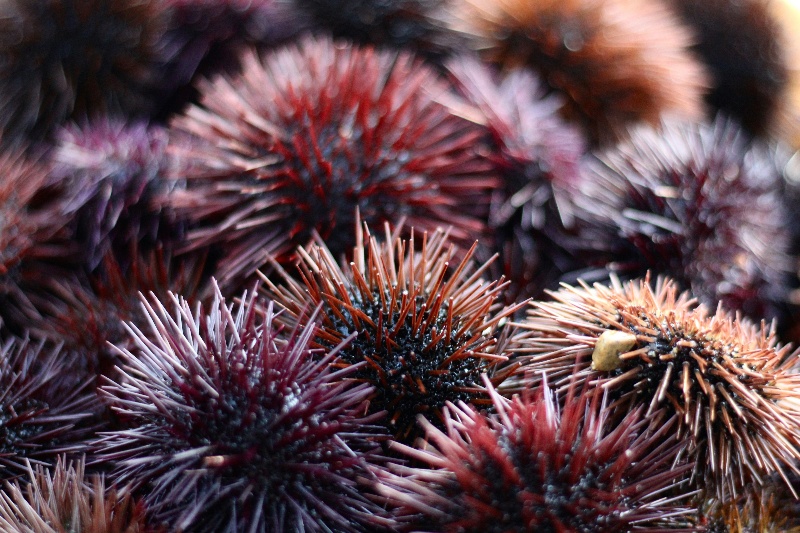
PI and co-PIs: Arie Spyksma (University of Auckland); Kelsey Miller (University of Auckland); Katerina Taskova (The University of Auckland); John Keane (University of Tasmania); Nicholas Perkins (University of Tasmania); Ariell Friedman (Greybits Engineering)
Funding amount: $131,000
Project overview: Climate change is leading to rapid changes in species distributions and corresponding changes to ecosystems. In Australia and New Zealand, climate-induced sea urchin population growth poses a significant threat to kelp-dominated reefs. To address the threat, this project aims to develop machine learning algorithms that can rapidly analyze underwater imagery to assess sea urchin expansion and kelp decline. This work will provide essential data for proactive ecosystem management and potentially serve as a foundation for similar projects worldwide.
Full abstract:
Click to expand
Kelp forests are among the most productive ecosystems on Earth, but climate-driven impacts are causing wide-spread kelp habitat loss, declines in biodiversity and reduced ecosystem services. The climate-driven range expansion of the longspined sea urchin, Centrostephanus rodgersii, is the most urgent threat to kelp-dominated reefs in south-eastern Australia. Rapid proliferation of urchin barrens has driven biodiversity losses, threatens economically important fisheries, and has downgraded the social, cultural and economic values of this vital ecosystem. Longspined sea urchins also threaten kelp forests in New Zealand; however, the extent of the damage is unknown. Responding to this threat by preventing sea urchin overgrazing can be effective and affordable but is currently unachievable in New Zealand due to data deficiencies. Assessing this specie’s impact requires the collection and analysis of underwater imagery spanning tens to hundreds of kilometres of reef. However, manual image annotation and analysis makes this process incredibly slow. The high contrast of sea urchins on barren reef makes this an ideal candidate for modern computer vision solutions based on machine learning (ML) algorithms to dramatically improve annotation and analysis, and provide timely information required for proactive management. Using existing image-based monitoring data we will develop ML algorithms to rapidly analyse the extent of kelp decline and barren expansion in New Zealand and make inference regarding the success of management actions in Australia. This project will generate ML algorithms that can be easily used, shared, and repurposed, laying the foundations for further climate related ML development within kelp forest ecosystems globally.
Oceans & Marine Systems Interpretable ML