The CityLearn Challenge 2023
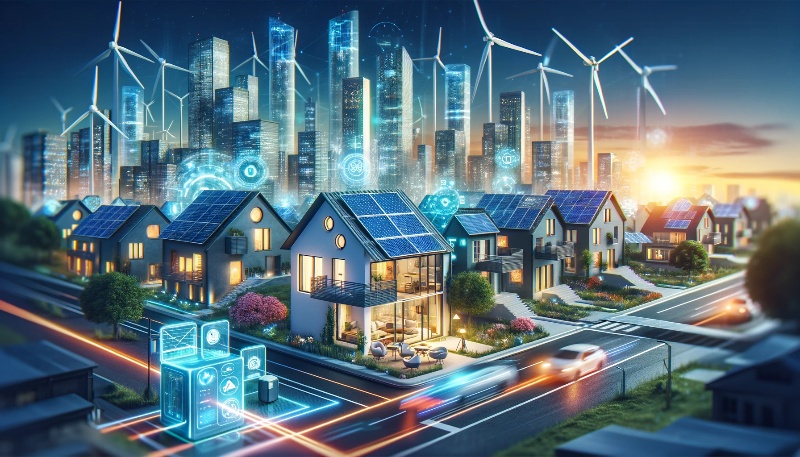
PI and co-PIs: Zoltan Nagy (The University of Texas at Austin); Javad Mohammadi (University of Texas at Austin)
Funding amount: $103,000
Project overview: The operation of buildings accounts for some 30% of global energy usage, making it an essential target for emissions reduction. This project, a novel edition of the popular CityLearn Challenge, leverages an easy-to-use simulator of neighborhood energy use to enable the scalable development and testing of advanced emissions-reduction algorithms. Specifically, the project makes possible the development of reinforcement learning-based control agents to decrease emissions from buildings at the neighborhood level, both by reducing energy use and by shifting operations to times when low-emissions electricity is available.
Full abstract:
Click to expand
The building stock is responsible for $30\%$ of greenhouse gas emissions. Thus reducing energy use and/or shifting operations to low emissions electricity is paramount to eliminate emissions from buildings. One of the main challenges for smart building energy controllers is to be able to be deployed with as little knowledge as possible and learn as quickly as possible to provide benefits. Meta-learning, transfer learning and offline learning have been proposed as potential solutions but their advantages are still unclear. In an attempt to tackle these problems, in this project we develop a representative model of neighborhoods, and execute a novel edition of the CityLearn Challenge. CityLearn is a OpenAI Gym Environment for the implementation of advanced control agents for demand response and reduction of carbon emissions at the neighborhood level, and one of the few environments suitable for this kind of study.
Cities & Urban Planning Buildings Reinforcement Learning