Unlocking Energy Flexibility of Residential Buildings
The Innovation Grants Program 2023 advances residential energy management with CityLearn
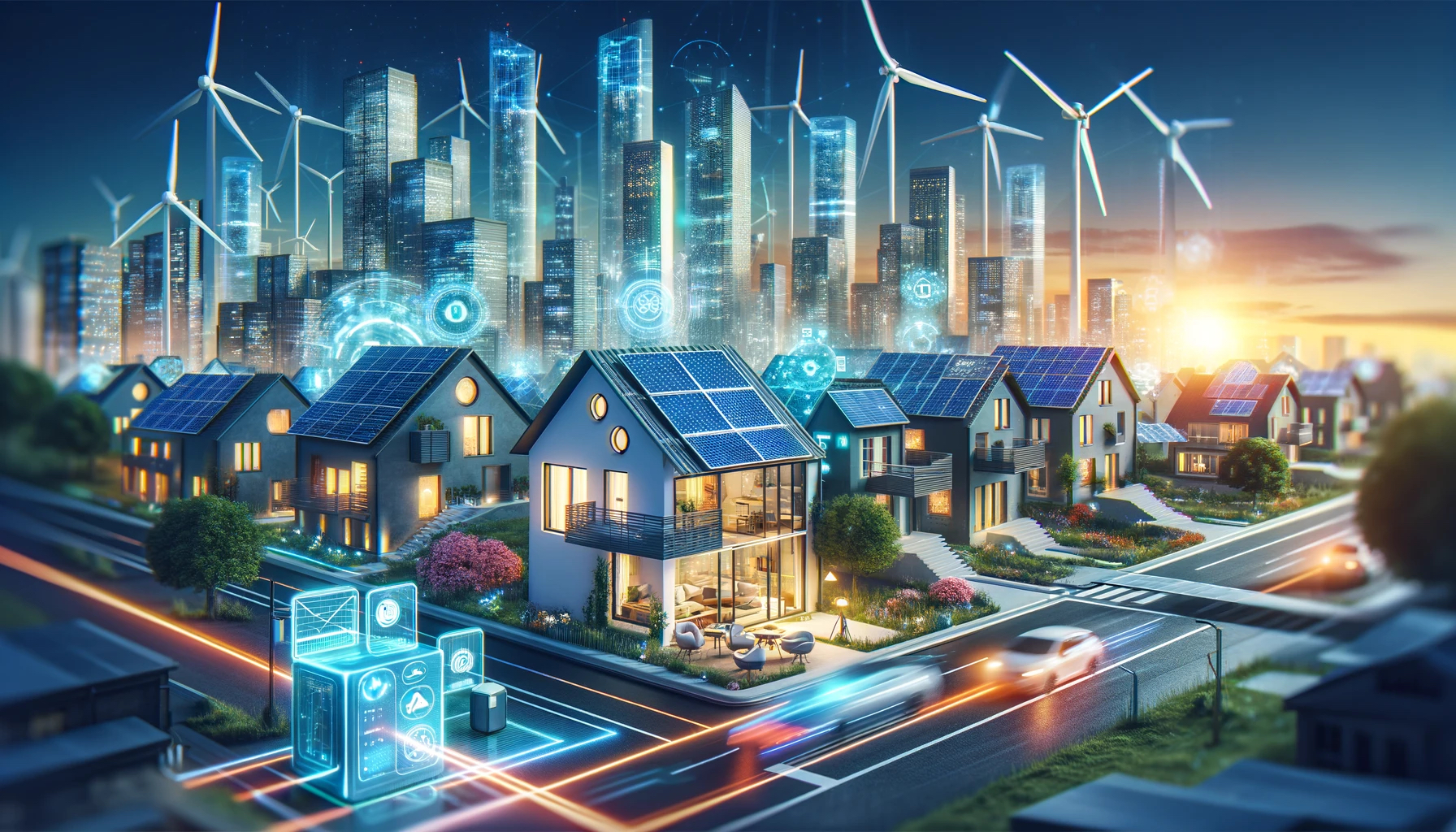
In the shift towards decentralized and renewable dominated energy grids, residential buildings play a crucial role. Their flexible demand is key to integrating renewables like solar and wind, which are crucial for a sustainable low-carbon future. Building energy management relies on energy forecasting, improving flexible demand, and scheduling charging/discharging of batteries and EVs, all areas that can leverage artificial intelligence and machine learning in the short and medium term.
Energy flexibility1 relies on energy users modulating or adapting their energy demand when needed, typically in response to a pricing signal or an emergency signal, e.g., to reduce air conditioning load on a hot afternoon. One under-utilized tool is continuous energy management, e.g., constantly adjusting loads as exemplified by smart home controllers.
The lack of a suitable benchmark environment has always been a bottleneck for developing such smart home controllers and highlighting their usefulness. Our CityLearn project is working to provide that benchmark environment.
Typically, smart home controllers focus on objectives like maximizing self-consumption or minimizing energy use or cost, with most projects designed as one-off developments. However, when buildings become part of the power grid infrastructure, integrating them becomes a multi-faceted and multi-objective problem, as their loads and potential PV generation, especially when aggregated, directly influence power generation needs. In addition, the design efforts are complicated by two ongoing challenges: integrating occupants and users into smart home energy controllers and simulation and understanding building loads as buildings increasingly electrify their current loads (e.g. space heating) and new loads are added at fast speeds (e.g. electric vehicle charging).
Here is where our CityLearn project has been at the forefront of development for the past several years. Instead of focusing on one particular aspect of demand-side management, we deliberately focused on integrating many distributed energy systems into a single computational environment to facilitate the development and benchmarking of advanced controllers. For example, we have shown that AI-based controllers can unlock energy flexibility by uncovering individualized occupant patterns and preferences, in a way that time-of-use pricing could not, leading to 10-15% reduced carbon emissions and >20% lower peak demand. In the same paper we found that a purely time-of-use controller did not reduce the aggregated peak at all, but merely shifted the peak load to a different time, which is unexpected at best. Similar unintended behaviors have been found by modulating smart thermostats, when all thermostats increase load at the same time due to their default setting.
Beyond these research findings, CityLearn has always been an open-source project; intended to be reused, updated, edited, and challenged by the community. We have held several versions of the CityLearn Challenge to crowdsource and accelerate innovation in this space. The most recent edition in 2023 has been made possible by the generous CCAI Innovation Grant.
In addition to the building control track which was included in previous years, we teamed up with Cambridge University’s Energy Efficient Cities Initiative which designed a forecasting track. The winners received a total of $5000 sponsored by Mitsubishi Electric Research Labs and presented their solutions at our NeurIPS 2023 Workshop. We have also created educational material, e.g., tutorials to get started quickly with CityLearn, for example at the CCAI Summer School. Most recently, we have integrated CityLearn into a course on Occupant-Centric Grid-Interactive Buildings.
The CCAI Innovation Grant 2023 also enables us to further push the capabilities of CityLearn, such as the development ofa methodology to integrate DOE’s recently released End-Use-Load-Profile database, which comprises representative models of all US buildings. This leap forward will allow us to estimate energy flexibility nationwide in the US. The methodology is generalizable to any location where archetypical building models exist and thus can be extended worldwide to facilitate the energy transition.
We are planning a major release of CityLearn in the coming weeks: in collaboration with Concordia University and the Politecnico di Torino, we are integrating occupant behavior models that can study how occupants interact with smart home controllers; and in collaboration with the Instituto Superior de Engenharia do Porto, we are integrating the much awaited EV module to study the impact that EVs can have on grid-aware demand side load management. These updates to the CityLearn platform move us closer to a vitally important, fine-grained computational environment to support emission reductions from buildings.
Thanks to CCAI’s generous support, we are getting closer to our vision for a more energy-flexible built environment, setting new benchmarks for researchers and practitioners.
The Climate Change AI Innovation Grants Program 2023 is supported by Quadrature Climate Foundation, Google DeepMind, Global Methane Hub, and the Canada Hub of Future Earth.
Notes
-
There are a lot of technical terms to describe energy flexibility which can almost be used interchangeably: demand side energy management, virtual power plants, load shedding/shifting, demand response, or demand dispatch ↩