NeurIPS 2023 Workshop: Blending new and existing knowledge systems
Find out more about CCAI’s NeurIPS 2023 workshop
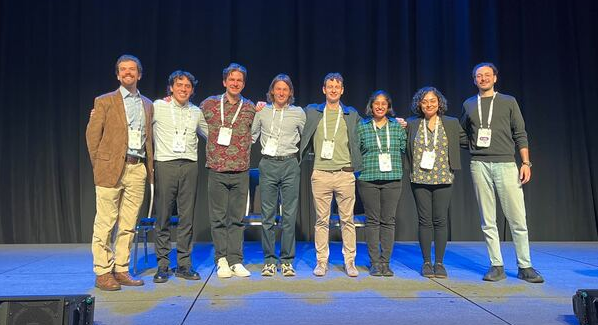
In December 2023, CCAI hosted a workshop at NeurIPS for the fifth consecutive year since 2019. NeurIPS is the largest conference in the AI field, today attracting thousands of participants across academia, industry, and government. The workshop brings together some of the latest research in AI that has potential to address the climate crisis. The 2023 iteration of the workshop focused on blending new and existing knowledge systems to inspire work that considers how novel machine learning research can build upon layers of institutional wisdom.
This year, 118 papers were accepted to the CCAI workshop, as well as 12 proposals and three tutorials. Of these, three papers were awarded prizes for “Best ML Innovation,” “Best Pathway to Impact,” and “Overall Best Paper.”
Overall Best Paper: Large Scale Masked Autoencoding for Reducing Label Requirements on SAR Data
In this paper, Allen et al. demonstrate a self-supervised approach to making satellite imagery (SAR data) classifiable during adverse weather conditions as well as night time hours. This advancement allows for remote intervention at rapid timescales during climate risk events. The novel breakthrough here was that the authors used SAR data (rather than typical RGB optical data), which can be detected at night and through adverse weather. SAR data is generated by satellites emitting microwaves down to Earth and then detecting the reflected waves to create a distinct image.
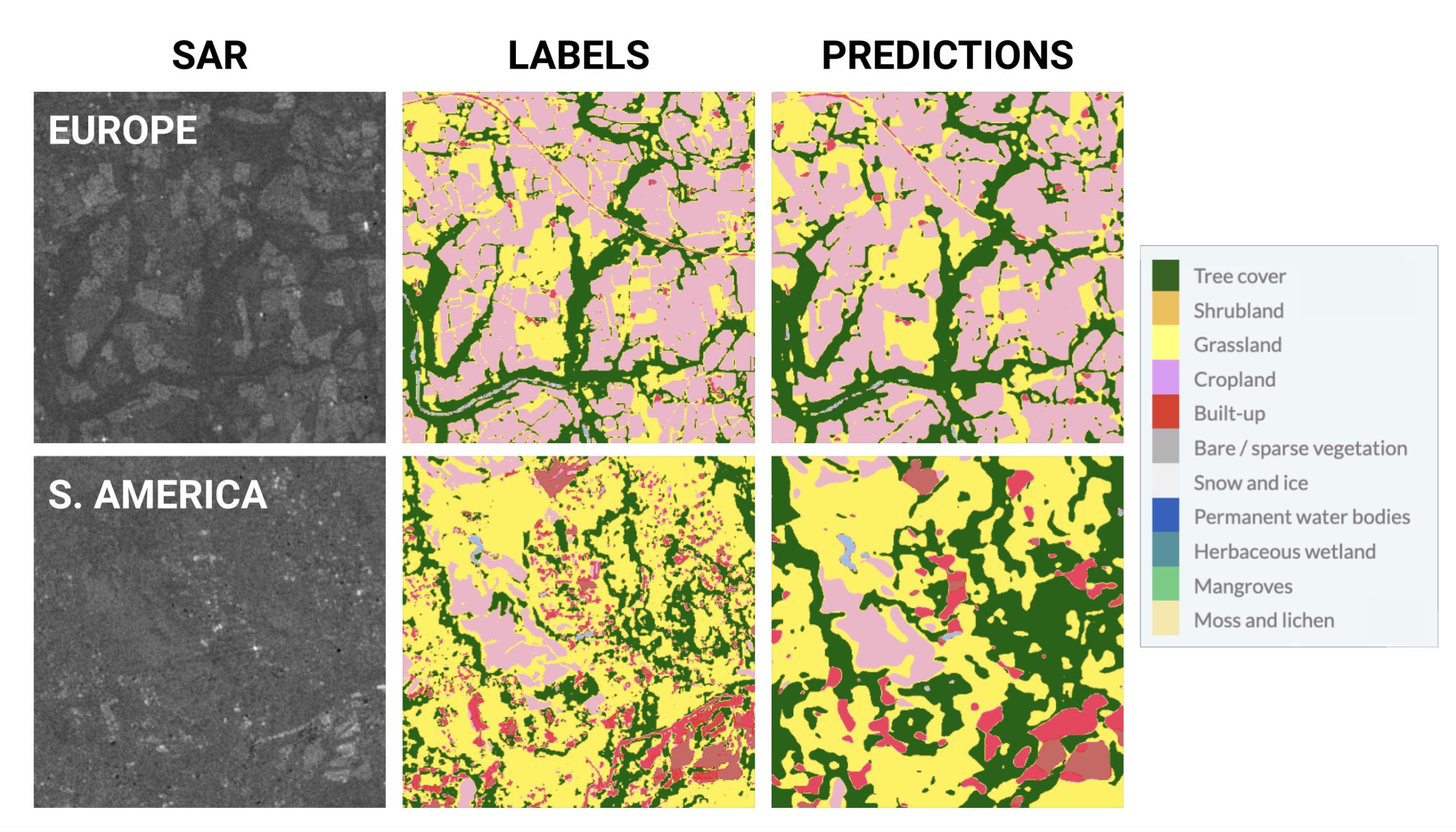
The authors used a self-supervised pre-training regime with a masked autoencoder to create a base model. They then fine-tuned the pre-trained model on two downstream tasks: a regression task (MODIS Vegetation Continuous Fields), and a classification task (ESA World Cover). It was found that the pre-training significantly helped with the downstream tasks. This pre-training reduces the need for expensive hand-labeled data, thereby making SAR a more attractive data source for remote sensing applications. Added to the fact that SAR data can be used in all weather conditions and at night, this makes this work particularly exciting in the remote sensing field.
Best ML Innovation: Real-time Carbon Footprint Minimization in Sustainable Data Centers with Reinforcement Learning
Sarkar et al. present a novel real-time data center control agent that minimizes carbon footprint, energy consumption, and energy costs. This is in the context of the World’s ever growing use of data centers, and growing energy intensity of data centers. Additionally, the paper takes into consideration battery storage optimization, HVAC energy, and flexible load of data centers. The authors present the use of a multi-agent reinforcement learning (MARL) agent, that achieves a 10% performance improvement across emissions reductions, cost, and energy savings.
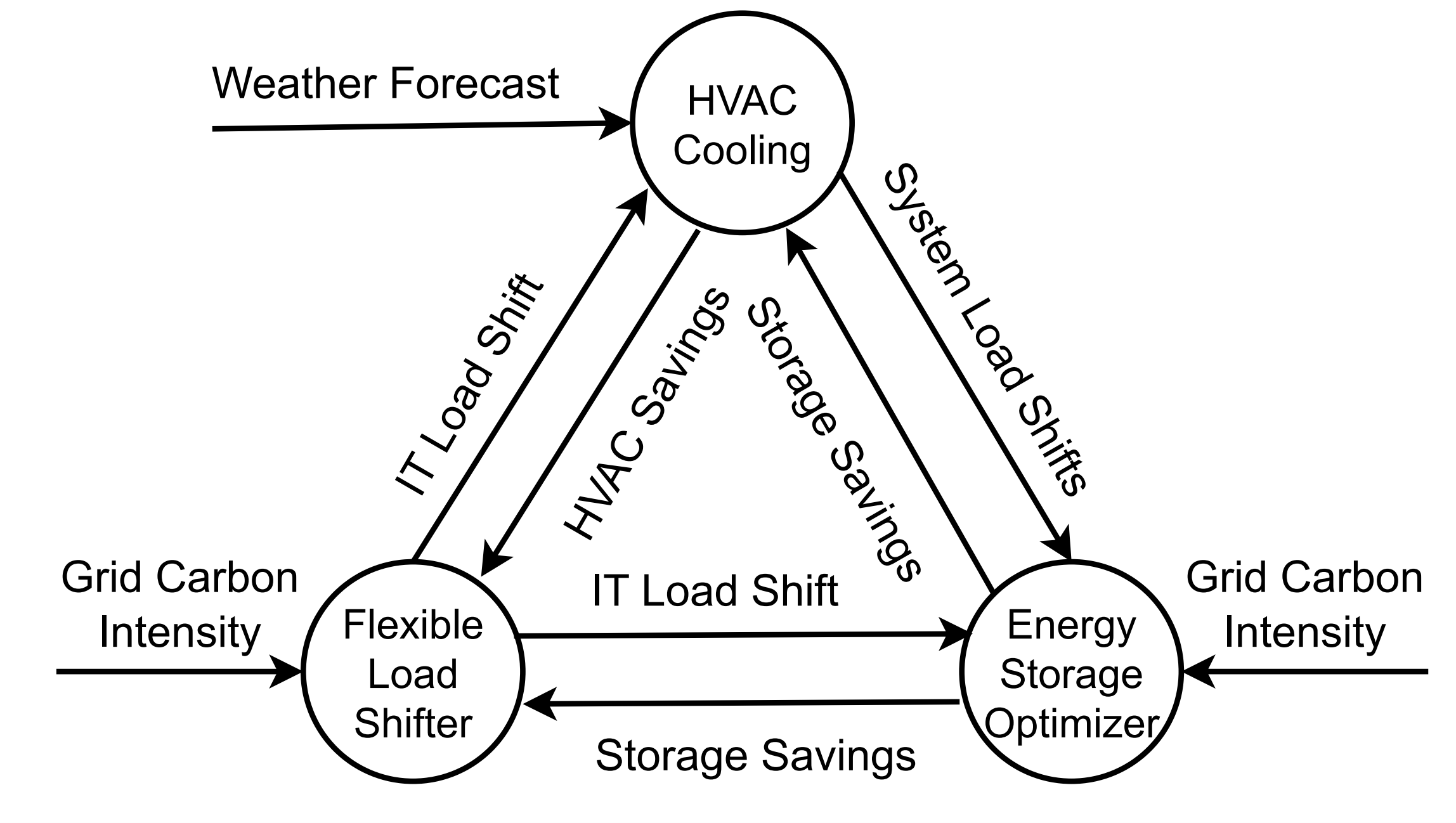
The authors plan to open source their DC-CFR framework to move the community forward in reducing carbon emissions of data centers. The reviewers particularly liked how clearly written the paper was, as well as the use of a novel method to target an important problem in the energy domain. Given the dramatic rise of data center usage (especially for AI training!), reducing the carbon intensity of their energy consumption will be key to helping us get to net zero.
Best Pathway to Impact: Discovering Effective Policies for Land-Use Planning
The global carbon balance is affected by both fossil fuel emissions and land emissions. These land emissions can vary widely depending on what the land is used for. For example, urban land typically releases more net carbon emissions than secondary forest vegetation (vegetation that has been touched by humans).
In this paper, the authors set out a framework for building a predictive model of land use change, that can then be used to evolve policies to find optimal policies. The authors combined data both from the land use harmonization project (LUH2) and from the sampling of the bookkeeping of land use emissions (BLUE) simulator. This training data set was used to build the surrogate model that was used by the prescriptor.
By searching through the solution space, the authors were able to construct a pareto front that trades off emissions reductions with land use change (the idea being that higher land use change might reduce economic impact). In doing so, policy makers can explore the optimal change, given a certain land use change tolerance they might have.
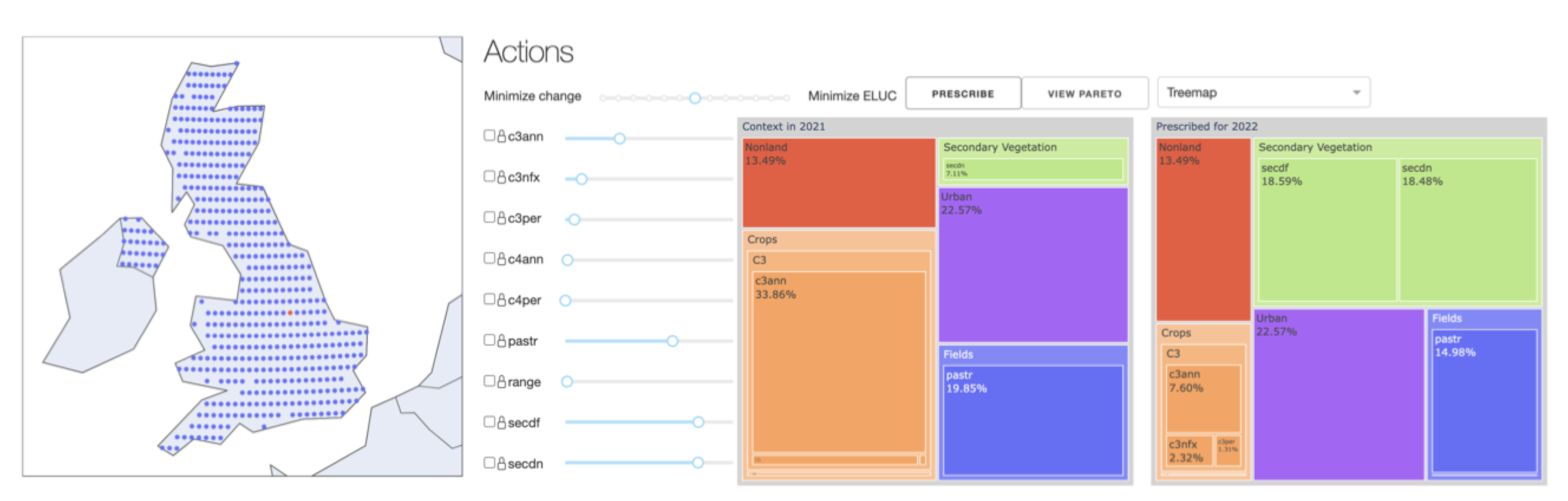
The authors also built a useful exploration and visualization tool, to help policy makers navigate the solution space. The tool is available at https://landuse.evolution.ml, and was valued by the reviewers as an important component to connect the work to stakeholders.
Tips for great workshop papers
The reviewers were grateful for all the submissions they received at this year’s NeurIPS workshop. Having so many submissions demonstrated the growing world of applying machine learning to address climate issues, and inspired the team with hope for our future. Among the winning papers, a few things stood out that made them great papers. Namely, these were that the papers were easy to read, linked the work to potential impact in the climate space, and placed the work in the context of previous research done in the area. The team at CCAI looks forward to workshops at future machine learning conferences, and would strongly encourage anyone thinking about submitting to do so.