Announcing the Climate Change AI Innovation Grants 2022 winners
13 proposals will be awarded a total of $1.8M USD as part of CCAI’s first research grants program
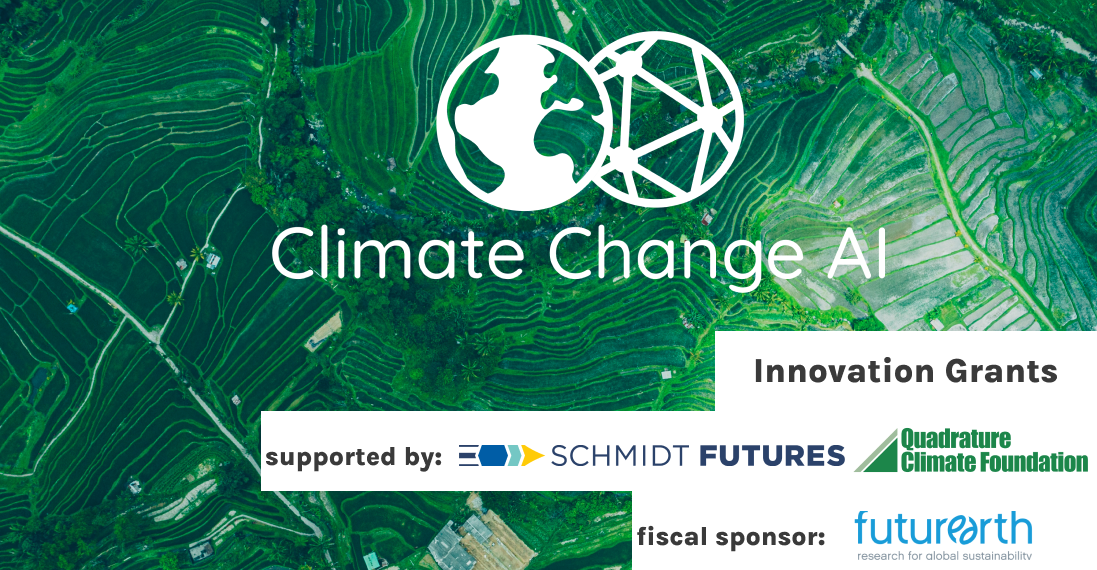
We at Climate Change AI are excited to announce the winners of our 2022 Innovation Grants program. These winners were selected through rigorous peer review by a range of experts in AI and climate change, and will receive USD 1.8M total split across thirteen project proposals.
The projects were chosen from almost 200 submissions from around the world, each proposing impactful, AI-driven approaches for addressing climate change mitigation and adaptation. The awarded proposals span a broad range of application areas, from energy to conservation, and investigators in six continents.
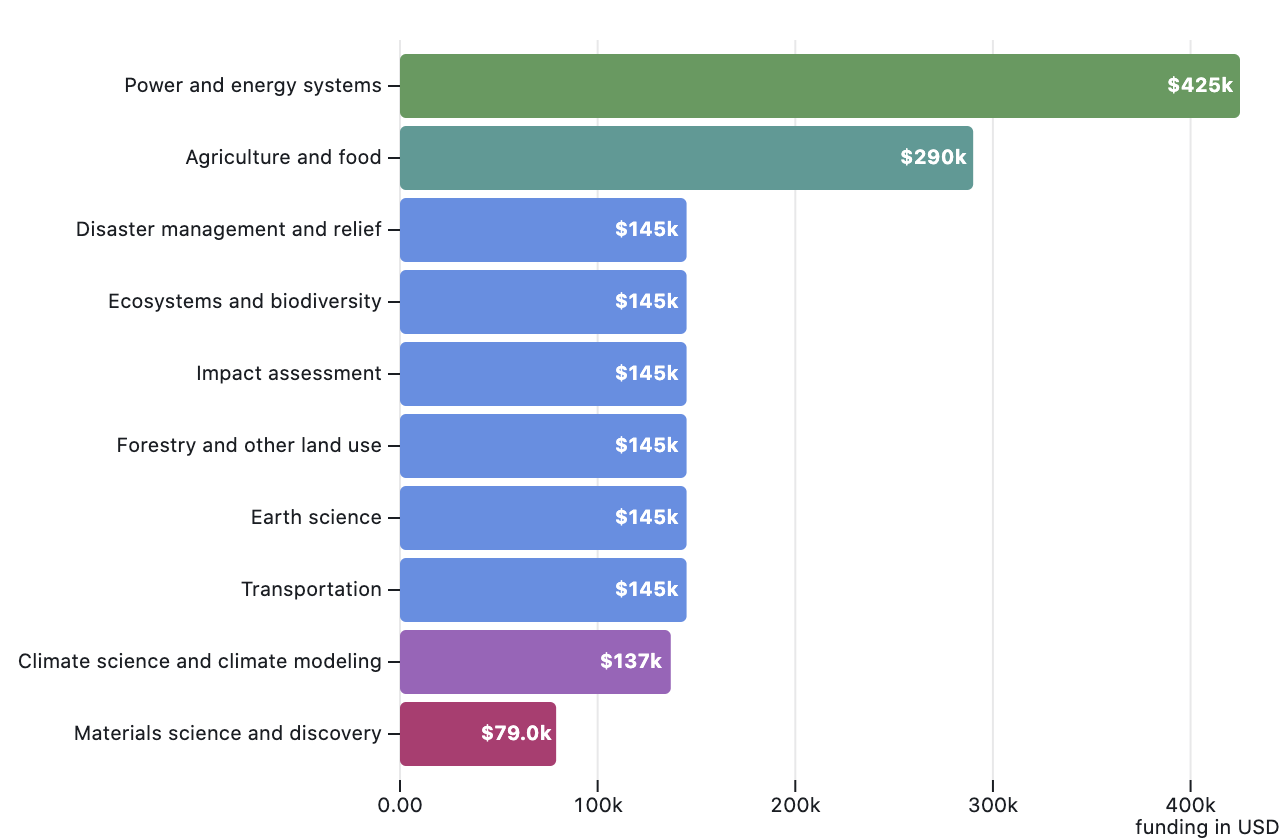
We were amazed and heartened by the high quality of the submissions to the Innovation Grants program. These proposals collectively represented significant potential impact for addressing climate change, and there were many high-quality proposals that we were unfortunately unable to fund. We’re therefore fundraising to expand the size of our funding program in the coming years, and hope that others will join us to help fill in the gaps.
For administrative reasons, the Innovation Grants were also limited to primary applicants from OECD countries (though teams could span multiple countries, including non-OECD countries). In future iterations of funding programs, we hope to drop this restriction and particularly fund applications from the Global South.
We’d like to thank Schmidt Futures and Quadrature Climate Foundation for their financial sponsorship of this program, and Future Earth for their significant administrative support.
The funded proposals are listed below (in alphabetical order):
![]() |
Accelerating Material Discovery for High-Performance Chemical Separation using AI Subhransu Maji (University of Massachusetts, Amherst); Peng Bai (University of Massachusetts, Amherst) |
![]() |
Adaptive Learning Techniques for Improved Subseasonal Forecasting Steve Easterbrook (University of Toronto); Judah Cohen (AER); Lester Mackey (Microsoft Research); Soukayna Mouatadid (University of Toronto); Genevieve E Flaspohler (MIT); Paulo Orenstein (IMPA); Ernest Fraenkel (MIT) |
![]() |
Detecting Flooding in Fiji’s Croplands John M Duncan (The University of Western Australia); Bryan Boruff (The University of Western Australia); Nathan Wales (The University of Western Australia); Solomoni Nagaunavou (Ministry of Agriculture, Fijian Government); Renata Varea (The University of the South Pacific); Kevin Davies (The University of Sydney); Eleanor Bruce (The University of Sydney) |
![]() |
Estimate the ice volume of all glaciers in High Mountain Asia with deep learning (ICENET) Niccolo Maffezzoli (Institute of Polar Sciences - National Research Council); Eric Rignot (University of California Irvine); Carlo Barbante (Institute of Polar Science - National Research Council) |
![]() |
Extracting and Discovering New Measurements from Climate Text Sources Taylor Berg-Kirkpatrick (University of California San Diego); Tom Corringham (Scripps Institution of Oceanography) |
![]() |
ForestBench: Equitable Benchmarks for Monitoring, Verification and Reporting of Nature-Based Solutions with Machine Learning Dava Newman (MIT); Moises Exposito-Alonso (Carnegie Institution for Science); Lucas Czech (Carnegie Institution for Science); David Dao (ETH Zurich); Björn Lütjens (MIT); Lauren Gillespie (Stanford University); Hilary Hao (Climate Reality Project); Andrew Cottam (Restor) |
![]() |
Improving Resiliency of Malian Farmers with Yield Estimation: IMPRESSYIELD Esra Erten (Istanbul Technical University); Ramazan Gokberk Cinbis (METU); Osman Baytaroglu; Traore Haoua (OKO Finance) |
![]() |
Learning Power System Dynamics in the Frequency Domain Baosen Zhang (University of Washington); Weiwei Yang (Microsoft Research); Yixing Xu (Breakthrough Energy) |
![]() |
Machine Learning-based Dynamic Climate Projections for Power System Planning Datasets Bri-Mathias S Hodge (University of Colorado Boulder); Aneesh Subramanian (University of California, San Diego); Claire Monteleoni (University of Colorado Boulder); Himanshu Jain (IIT Roorkee) |
![]() |
Matching Structured Energy System Data for Policy Making and Advocacy using Weakly Supervised Machine Learning Xu Chu (Georgia Tech); Zane Selvans (Catalyst Cooperative) |
![]() |
Mitigating Climate Change Impacts on Biodiversity via Machine Learning Powered Assessment Oisin Mac Aodha (University of Edinburgh); Scott Loarie (iNaturalist); Thomas Brooks (IUCN) |
![]() |
Towards greener last-mile operations: Supporting cargo-bike logistics through optimized routing of multi-modal urban delivery fleets Maria S Astefanoaei (IT University of Copenhagen); Akash Srivastava (MIT–IBM Watson AI Lab); Kai Xu (The University of Edinburgh); Nicolas Collignon (Kale Collective); Esben Sørig (Kale Collective); Soon Myeong Yoon (Kale Collective) |
![]() |
Using Machine Learning and Earth Observation Data to Identify Aquaculture Sites with High Potential for Production Intensification and Mangrove Restoration in Southeast Asia Jack Kittinger (Arizona State University); Dane Klinger (Conservation International); Pia Faustino (Thinking Machines Data Science) |
The icons used in the table above are obtained via remixicon.com.