Accelerating Material Discovery for High-Performance Chemical Separation using AI
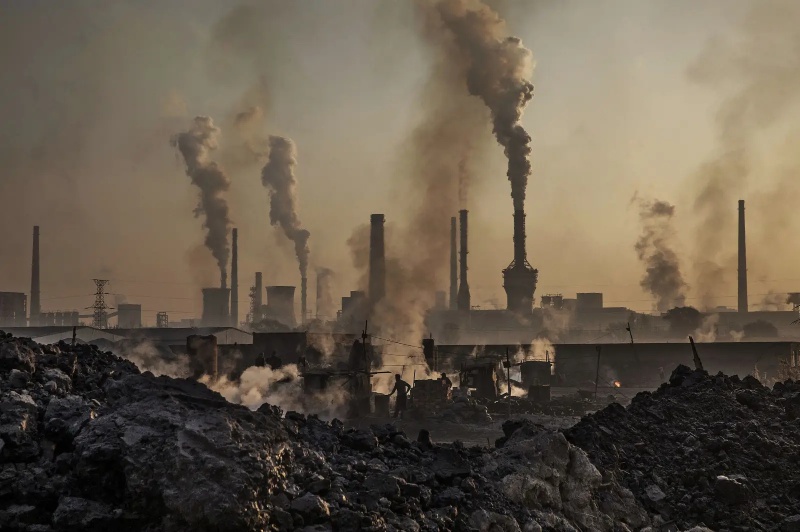
PI and co-PIs: Subhransu Maji (University of Massachusetts, Amherst); Peng Bai (University of Massachusetts, Amherst)
Funding amount: $79,000
Project overview: Chemical separation processes account for over 10 percent of global energy consumption and play a pivotal role in carbon capture and sequestration. By using novel machine learning-driven methods for the discovery of new, improved chemical separation processes, global energy consumption can be reduced, and the efficiency of carbon capture and sequestration technologies can be enhanced. This project aims to develop AI methods for discovery of materials that can improve separation processes in climate-critical applications. By tackling the energy-intensive nature of traditional separation processes, this project aims to offer innovative climate solutions in the petrochemical and carbon capture sectors.
Full abstract:
Click to expand
Separation of chemical mixtures accounts for more than 10% of global energy consumption, driven heavily by thermal processes such as distillation. Replacing these energy-intensive traditional separation processes with more efficient alternatives is projected to eliminate 100 million tonnes of CO2 emission and save billions of dollars in energy costs. Among the alternatives, separation using nanoporous materials, for example in an adsorption or membrane-based setup, can be an order of magnitude more efficient. These separation techniques are also considered as key next-generation technologies to enable cost-effective carbon capture and sequestration. Despite their great promise, identifying the optimal material out of the large pool of candidate structures for a given separation task requires significant resources and prolongs the development cycle. The proposed research aims to develop AI techniques for mitigation approaches in the chemical sector by predicting optimal materials for targeted separation applications. We will study representation learning, transfer learning, and active learning tasks for a series of separation applications. We will focus on primarily nanoporous zeolites, for which we already have several existing datasets and the capability to generate a new dataset relevant for CO2 capture. Broader outcomes of the proposed work include 1) a suite of machine-learning descriptors and benchmarks of their performances for representing extended materials structures and 2) appropriate methods to account for uncertainties in training data as well as the technical know-how to address the data accuracy-acquisition cost and exploitation-exploration trade-offs in building a materials AI system for chemical separations.
Chemistry & Materials Carbon Capture & Sequestration