Deep learning of nanoporous materials for chemical separations
With support from the Climate Change AI Innovation Grants program, an interdisciplinary team from UMass Amherst is using AI to help researchers design energy-efficient separation processes.
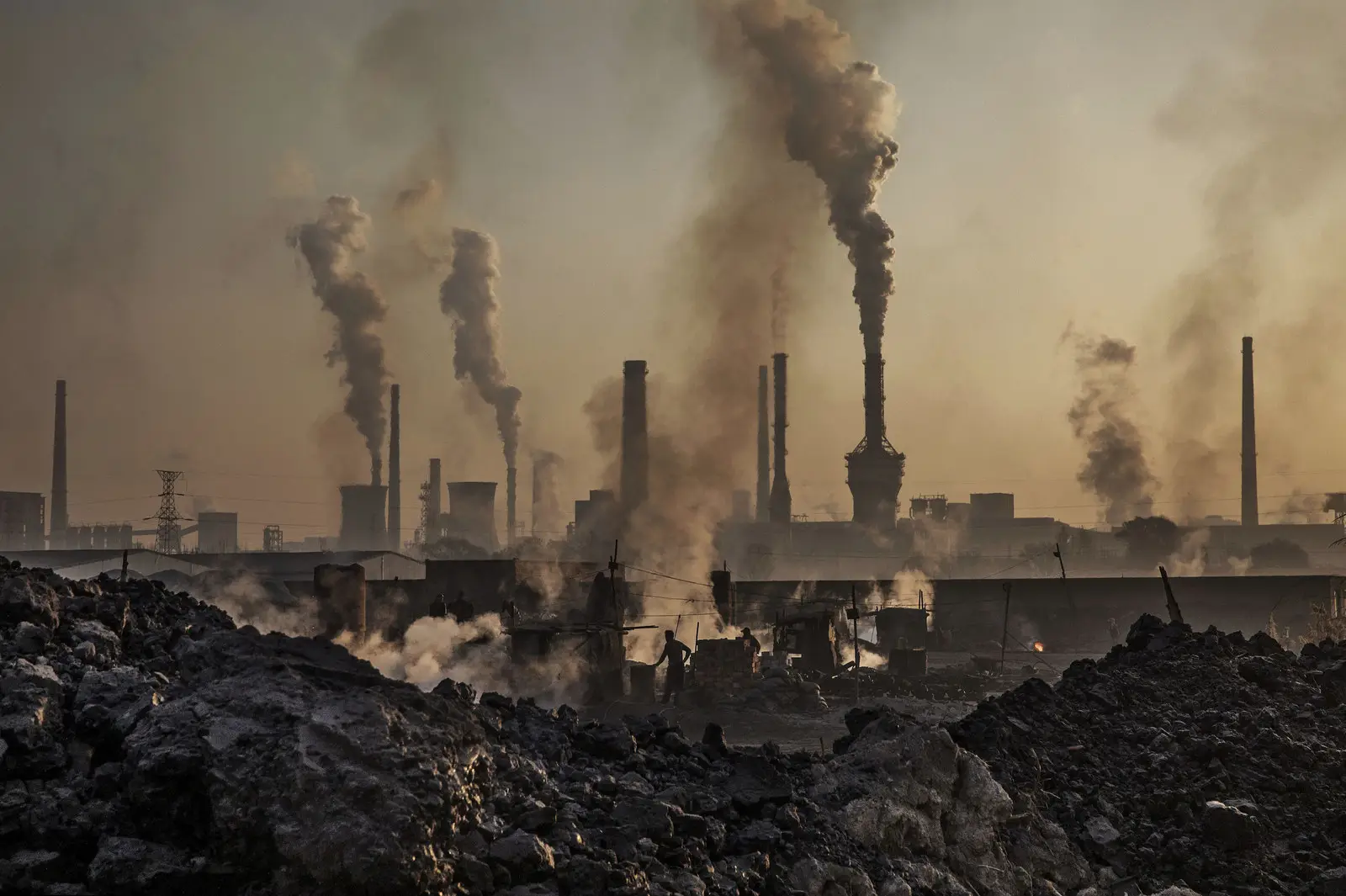
Separations are foundational processes in the chemical industry, accounting for about half of US industrial energy use and more than 10% of the world’s total energy consumption. An analysis of the largest energy consuming industries indicate that replacing traditional separation processes with more efficient alternatives could potentially eliminate 100 million tonnes of carbon emissions and save billions of dollars in energy costs annually. Separation also serves as a cornerstone of carbon capture and storage, enabling the selective removal of carbon dioxide from pre- and post-combustion gas at power plants, as well as from the environment using emerging direct air capture technologies.
Separation using nanoporous materials — which function as molecular sieves to allow certain molecules to pass through while rejecting others (see Figure 1) — can be an order of magnitude more efficient than heat-driven distillation processes (i.e., boiling liquids to obtain separate fractions at different temperatures). However, designing nanoporous materials involves identifying the optimal material structure for a target application, which can be challenging due to the large number of possible materials to choose from.
Among the porous materials that could be used for separation, zeolites stand out as robust and industrially proven. To date, hundreds of thousands of potential zeolite structures have been computationally predicted. However, so far, only about 250 have been synthesized experimentally and even fewer are used for practical applications. Despite their great promise, testing even the hundreds of currently synthesizable structures would require an impractical amount of effort and resources.
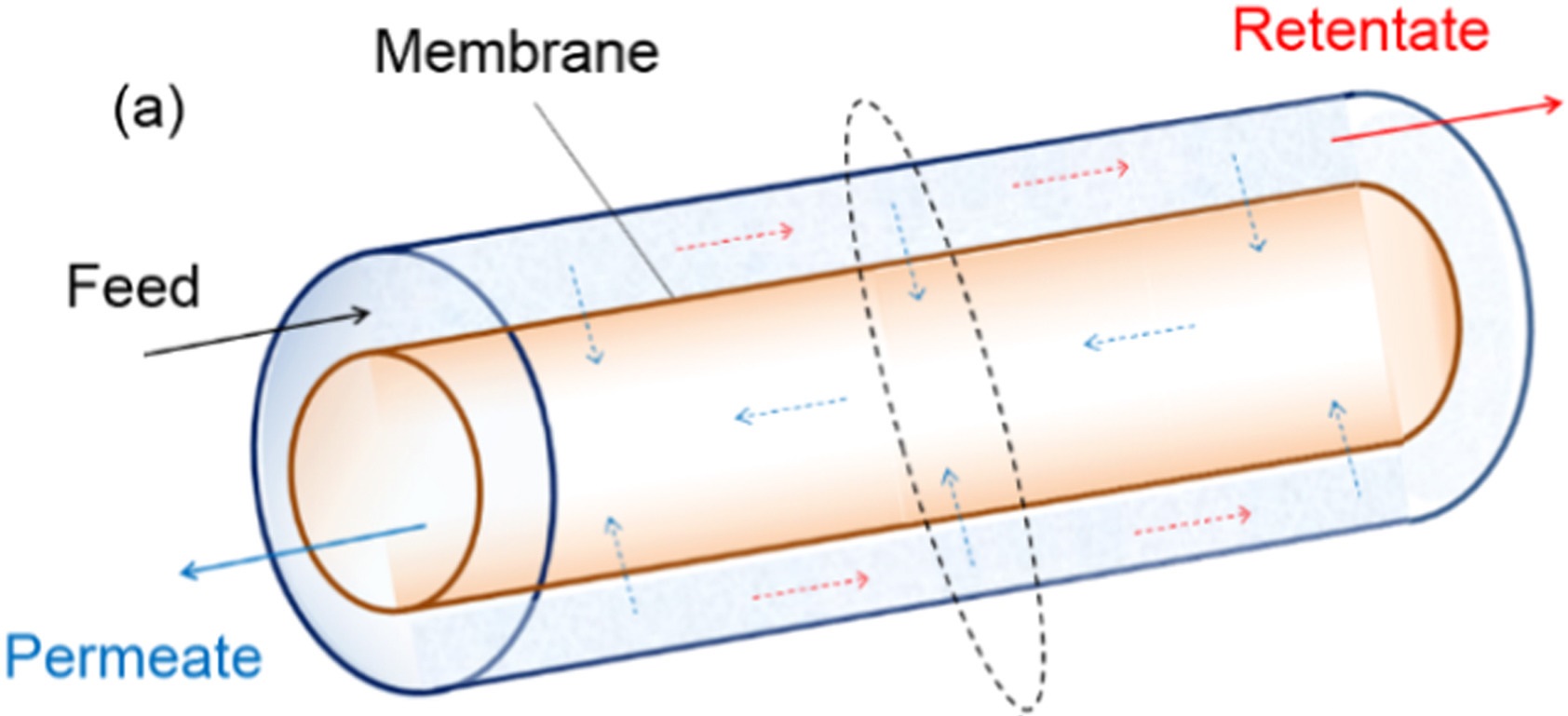
In this project, a deep learning framework named ZeoNet was developed to rapidly and accurately predict the separation performance of zeolite materials for large, flexible molecules (see Figure 2). ZeoNet borrows ideas from the computer vision community, viewing materials as 3-dimensional images. On a standard GPU, ZeoNet can process more than eight structures per second. This speed is in stark contrast to state-of-the-art computer simulations that can take hours per structure and lab experiments that can take at least days. ZeoNet also generalizes to novel structures not seen in the training set.
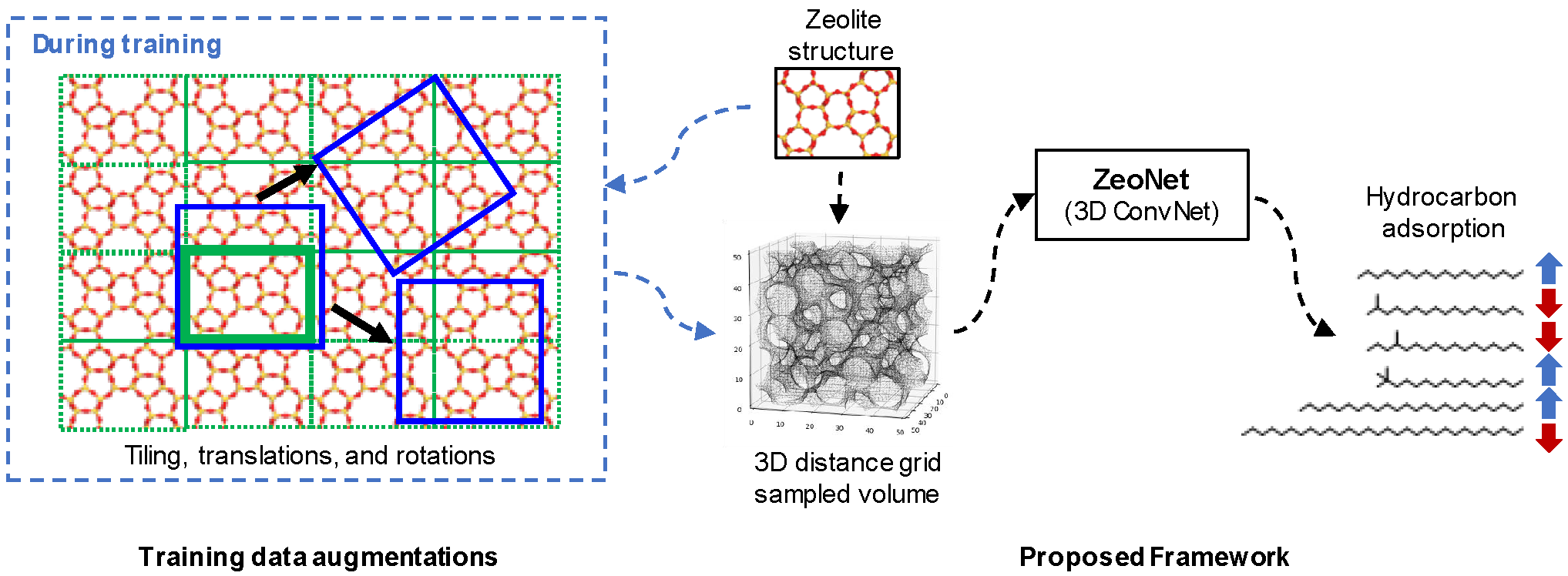
Preliminary follow-up work also shows that ZeoNet, once trained on one system (i.e., to predict adsorption of a specific molecule), can be easily adapted to work for another application with significantly less training data (with as few as ~500 training samples). This suggests that the ZeoNet model captures a rich, universal representation of the 3-dimensional structure of zeolites. What’s particularly exciting is that this representation appears to allow highly specific discrimination about which computer predicted structures might actually be synthesizable — a grand challenge in zeolite science. Collaborations with experimental groups are currently underway to test these ideas in physical labs.
This work is published as a featured article in the Journal of Materials Chemistry A and we are also releasing the dataset and software code in the hope of encouraging more AI researchers to work on physical sciences and engineering problems.
The Team
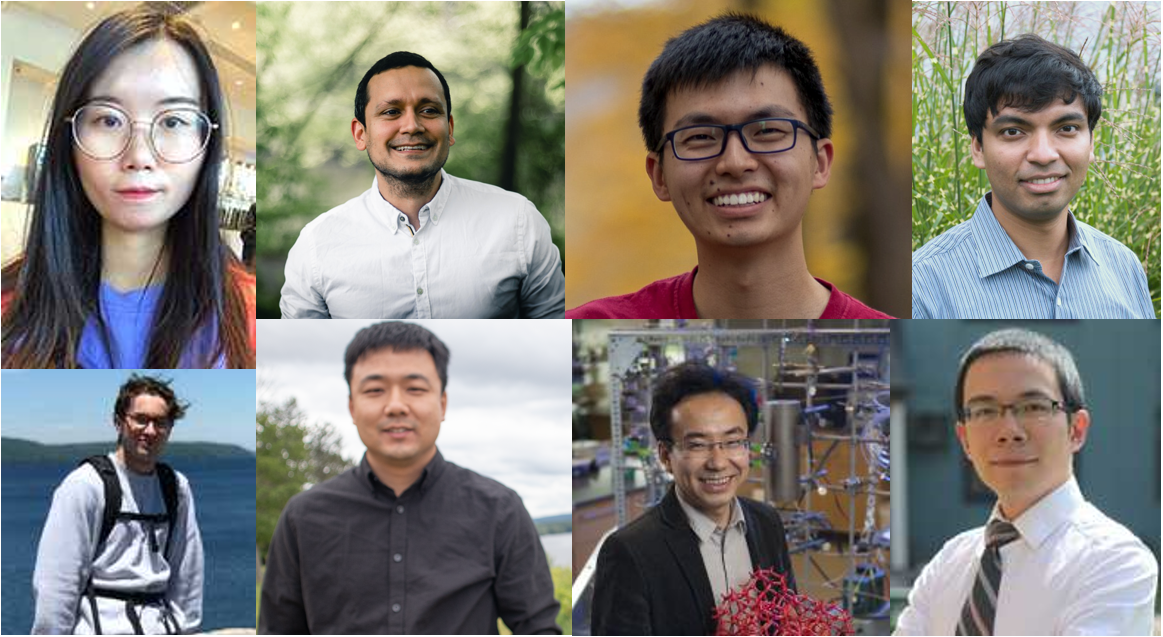
ZeoNet was developed in collaboration between the College of Engineering led by PI Peng Bai and his students Yachan Liu and Samuel Hoover, as well as Prof. Wei Fan and the College of Information and Computer Sciences led by PI Subhransu Maji and his students Gustavo Perez, Aaron Sun, and Zezhou Cheng at University of Massachusetts, Amherst.