Improving Resiliency of Malian Farmers with Yield Estimation: IMPRESSYIELD
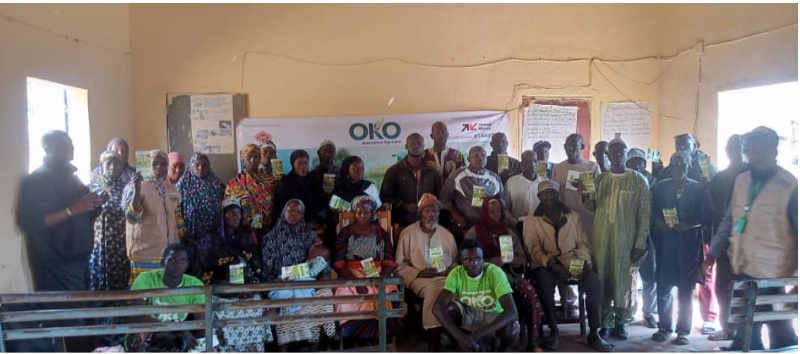
PI and co-PIs: Esra Erten (Istanbul Technical University); R. Gökberk Cinbiş (Middle East Technical University); Dr. Traore Haoua (OKO Finance Limited); Osman Baytaroğlu (Agcurate Bilgi Teknolojileri Anonim Şirketi)
Funding amount: $145,000
Project overview: Four million people in Mali depend on the cotton industry for their livelihoods, but cotton production faces significant risks from climate change, including shorter growing periods and extreme weather. Mali ranks low in resilience to climate change, positioned 169th out of 181 countries on the ND-GAIN index. This project aims to improve the resilience of cotton growers in Mali via machine learning-assisted yield estimation, using Earth observation data to monitor the temporal and spatial dynamics of cotton production. By combining high-resolution synthetic aperture radar data with on-the-ground labeled data, the team aims to predict evapotranspiration and thus estimate cotton yield at scale. Such estimates can reduce the vulnerability of Mali’s agricultural communities to climate change and support community welfare.
Full abstract:
Click to expand
One-quarter of Malians’ livelihood depends on cotton production, which is the largest agricultural export of Mali. However, Mali’s cotton production is at high risk due to the expected shorter growing periods, increasing duration of extreme temperatures, more frequent and intense droughts and rainfalls due to the climate change.A first step towards adaptation to future climate change is reducing vulnerability and exposure to present climate variability and insurance, specifically index-insurance, is a major instrument which can foster the adaptation. In this context, a key but unanswered question is achieving high accuracy field level yield estimations that can be used for designing yield-index insurance products within environments where in-situ data is scarce and/or untraceable and those EO products, associated with cotton yield, namely evapotranspiration (ET) and vegetation indices are limited temporally due to the high cloud fraction. The objective of the “IMProving RESiliency of Malian farmerS with YIELD estimation” (IMPRESSYIELD) project is then to deliver an innovative yield estimation method at commune scale and field scale for cotton, with a focus on the case study in Mali. We propose to build a deep SAR driven high-resolution ET approximation model, where approximately weekly estimates can be obtained via Sentinel-1 imagery, independent of weather conditions. The main challenge is the lack of high-resolution ET ground truth values needed for training purposes and we propose a novel weakly supervised training approach that aims to leverage low-resolution ET estimates that can be obtained on favourable weather conditions. The resulting high-resolution ET estimates will be used in a ML based field scale yield estimation model, which is trained by commune level historical yield averages. We believe that output field level yield estimations can be a direct input to yield-index insurance products, ensuring climate resiliency for smallholder farmers.
Climate Finance & Economics Agriculture & Food Earth Observation & Monitoring Societal Adaptation & Resilience