Matching Structured Energy System Data for Policy Making and Advocacy using Weakly Supervised Machine Learning
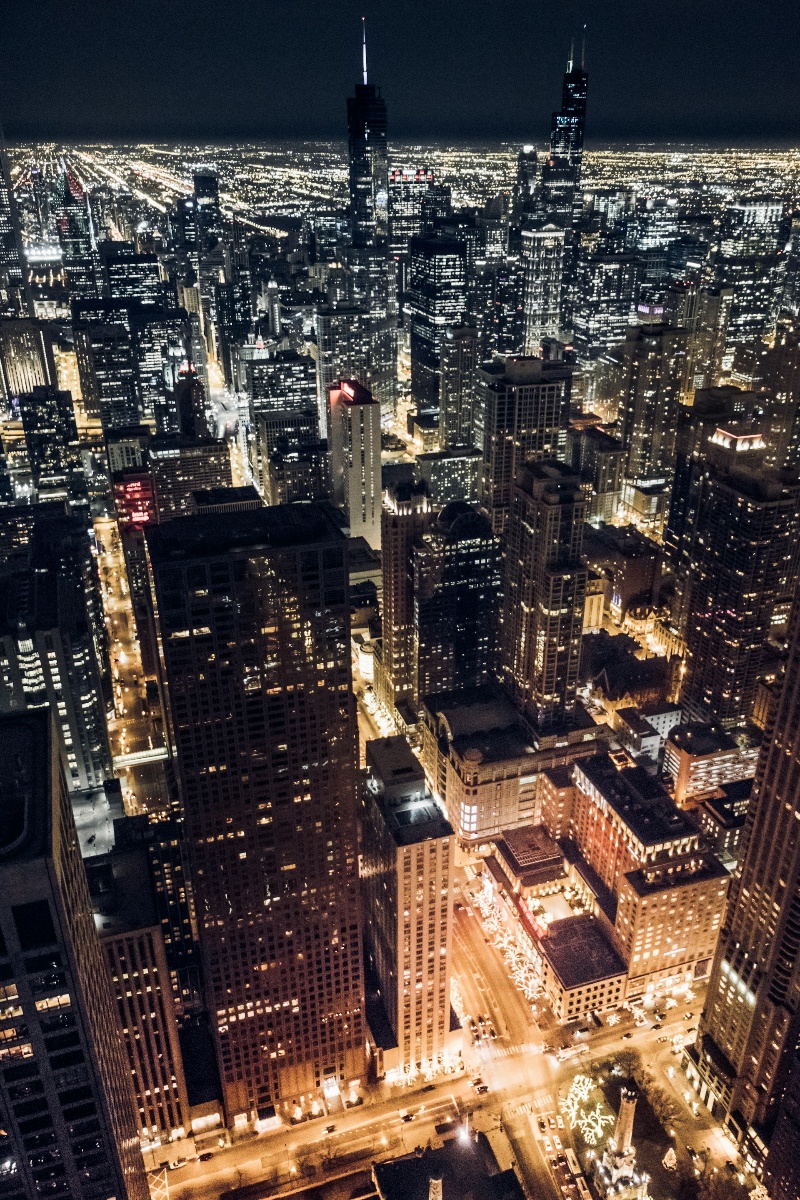
PI and co-PIs: Xu Chu (Georgia Tech); Zane Selvans (Catalyst Cooperative)
Funding amount: $145,000
Project overview: The accessibility of energy systems data is crucial for policy-making and climate advocacy, but data are currently scattered across multiple different reporting structures. Typically, working with diverse data sources has involved significant manual labor to find, reformat, and align relevant information. This project develops open-source software using machine learning to perform these tasks automatically for information within the US energy system. Such software will make it radically easier for advocates to make compelling cases for the economic benefits of the energy transition, and for policymakers to shape data-driven decisions.
Full abstract:
Click to expand
Transitioning from fossil fuels to renewable energy relies on climate advocates' ability to make compelling data-driven arguments. This ability is frequently limited by a lack of analysis-ready data describing both the financial and operational characteristics of the existing energy system. While these relevant public data are available, the financial and physical information about identical generators, power plants, and utilities are reported to different government agencies, and they often lack any shared identifiers that can be used to easily join these data together. Analyzing these aspects of energy systems in tandem has historically required a laborious process of joining related tables by hand before they can be used to guide legislation or produce expert testimony for use in regulatory proceedings. This work aims to greatly reduce the manual work required to integrate energy system financial data reported to the US Federal Energy Regulatory Commission (FERC) with the physical energy system data reported to the US Energy Information Administration (EIA). In particular, this work proposes to develop a weakly supervised machine learning system that innovates by (1) providing an easy-to-use interface to solicit user input in the form of programmatic labeling functions; and (2) designing a novel constrained probabilistic graphical model to combine available noisy and correlated user input while incorporating EM application specific matching constraints. The final system will not only enable high-quality integration of electric utility data with many pathways to impact, but will also save substantial human effort for integrating natural gas utility data that presents many of the same challenges and opportunities.
Power & Energy Public Policy