Adaptive Learning Techniques for Improved Subseasonal Forecasting
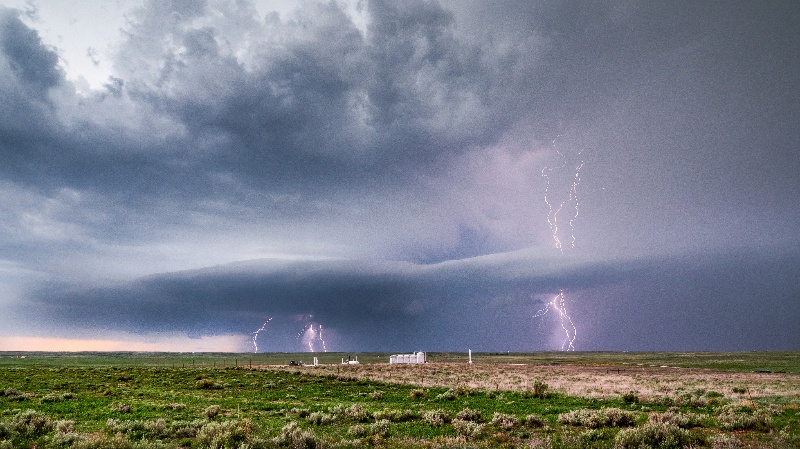
PI and co-PIs: Steve Easterbrook (University of Toronto); Judah Cohen (AER); Lester Mackey (Microsoft Research); Soukayna Mouatadid (University of Toronto); Genevieve E Flaspohler (MIT); Paulo Orenstein (IMPA); Ernest Fraenkel (MIT)
Funding amount: $137,000
Project overview: Subseasonal weather patterns fall in between long-term climate trends and short-term weather events, and subseasonal weather forecasting is an essential tool in responding to droughts, heightened wildfire risk, and other impacts of climate change. This project combines machine learning with standard approaches to subseasonal weather forecasting, via adaptive bias correction. Such methods have the potential to outperform both traditional methods and pure machine learning. The team aims to hone their algorithmic toolkit on US regions before expanding to support subseasonal forecasts at a global scale.
Full abstract:
Click to expand
Water and fire managers rely on subseasonal forecasts 2-6 weeks in advance to allocate water, manage wildfires, and prepare for droughts and other weather extremes. However, skillful forecasts for the subseasonal regime are lacking due to the complex dependence on both local weather and global climate variables and the chaotic nature of weather. To address this need, we propose to use machine learning, to develop an adaptive bias correction method, which augments traditional subseasonal benchmarks with simple but effective learned enhancements. Our improved benchmarks will include: (a) Climatology++, an adaptive alternative to climatology; (b) ECMWF++, a learned ECMWF correction; and (c) Persistence-ECMWF++, an augmented persistence model that combines ECMWF forecasts with lagged measurements. As an initial test, we have developed analogous models based on the U.S. operational Climate Forecasting System version 2 (CFSv2) and found them to outperform both operational practice and state-of-the-art machine learning and deep learning forecasters when applied to temperature and precipitation forecasting in the contiguous U.S. In this project, we will develop more accurate models based on the state-of-the-art ECMWF dynamical model, extend these forecasts from regional U.S. forecasts to global forecasts, apply adaptive online learning to ensemble the individual benchmark predictions, and generate both deterministic and probabilistic subseasonal temperature and precipitation forecasts. We anticipate the insights and improvements we propose will benefit both researchers and practitioners and plan to release our model code through the s2s_toolkit Python package and our routinely updated SubseasonalClimate dataset to facilitate future subseasonal benchmarking and development.
Climate Science & Modeling