ForestBench: Equitable Benchmarks for Monitoring Verification and Reporting of Nature-Based Solutions with Machine Learning
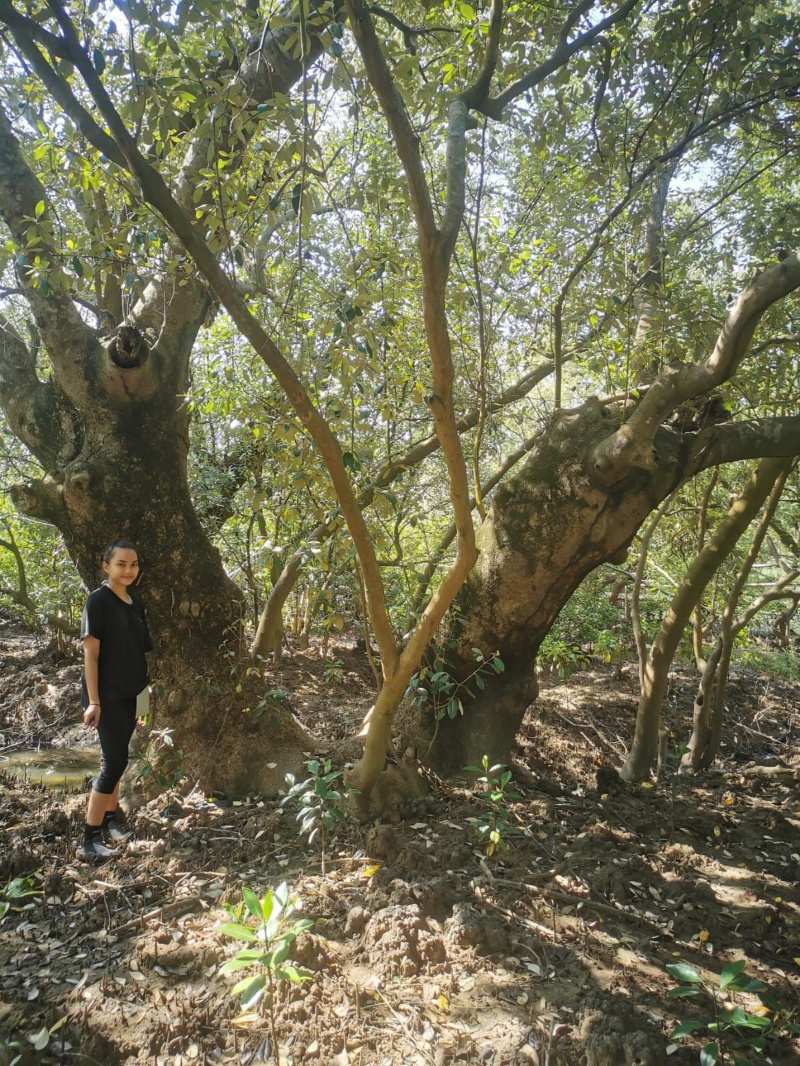
PI and co-PIs: Dava Newman (MIT); Moises Exposito-Alonso (Carnegie Institution for Science); Lucas Czech (Carnegie Institution for Science); David Dao (ETH Zurich); Björn Lütjens (MIT); Lauren Gillespie (Stanford University); Hilary Hao (Climate Reality Project); Andrew Cottam (Restor)
Funding amount: $126,290
Project overview: Over the last twenty years, the world has lost an area of forest equivalent in size to Europe, with massive implications for both carbon sequestration and biodiversity. Mapping forest carbon stock is essential to informing land use policies, as well as incentivizing land custodians via monitoring, reporting, and verification coupled to carbon offset systems. This project aims to advance the use of machine learning for carbon stock estimation in forests, by gathering data on carbon stock from high-priority areas for forest preservation across the Global South, in partnership with local and indigenous communities. The team aims to use this data to run a machine learning benchmark competition to improve the quality of algorithms for carbon stock estimation. Such work stands to improve geographical equity in forest preservation, and better equip local communities, as well as national and international policymakers, with the tools and incentives needed to advance nature-based climate change solutions.
Full abstract:
Click to expand
The deterioration of the natural world is unparalleled in human history and a key driver of the current climate crisis and global extinction. In the past twenty years, we have lost forest area equivalent to the size of Europe, accounting for 18% of global anthropogenic emissions. Reducing deforestation, restoring ecosystems, and natural sequestrating of carbon is therefore of uttermost importance and urgency. As recent research has shown, current manual measurements systematically overestimate forestry carbon credits. There is thus need for higher-quality carbon offsetting protocols and more transparency and accountability in the Monitoring, Reporting, and Verification (MRV) of the forest carbon stock. In recent years, remote sensing and Machine Learning (ML) have been used to estimate biomass based on drone and satellite data. However, these algorithms risk additionally contributing to the systematic overestimation of carbon stocks, unless they are properly calibrated and transparently validated. This proposal aims to collect and curate geographically-balanced gold-standard datasets of small-scale forest plots in the global south. In particular, we propose to collect field-based ground-level measurements of tree biomass, and drone-based imagery of tree canopies, in order to develop equitable benchmarks for ML-based MRV. The southern hemisphere is underrepresented in the available data; we hence aim to support local and Indigenous communities by collaborating with them for data collection. Furthermore, we plan to organize an ML benchmark competition to audit existing and novel models for MRV. The collected aerial images will be used by the participants of the competition to estimate biomass, while the field data will serve as ground truth for the evaluation.
Ecosystems & Biodiversity Forests Earth Observation & Monitoring Local and Indigenous Knowledge Systems