Estimate the ice volume of all glaciers in High Mountain Asia with deep learning (ICENET)
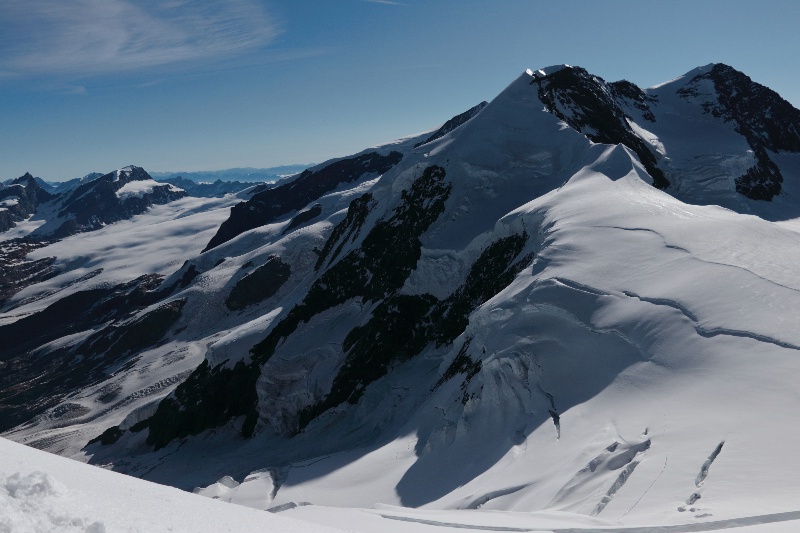
PI and co-PIs: Niccolò Maffezzoli (Institute of Polar Sciences – Italian National Research Council, CNR-ISP); Eric Rignot (University of California, Irvine, UCI); Carlo Barbante (Institute of Polar Sciences – Italian National Research Council, CNR-ISP)
Funding amount: $145,000
Project overview: Melting glaciers pose a significant threat to global sea level, biodiversity, and the 1.9 billion people who depend on glacier-sourced freshwater. This project aims to use AI-based remote-sensing algorithms to estimate the ice volume of glaciers across all of High Mountain Asia, encompassing over 80,000 individual glaciers. Specifically, state-of-the art image inpainting methods are applied to satellite-based digital elevation models, alongside gravity and ice surface velocity data, in order to infer subglacial topography and thus ice volume. The resulting dataset promises to be a vital tool for both climate change adaptation and Earth science, while the methodology developed may prove useful in other regions as well.
Full abstract:
Click to expand
Estimating the ice volume of Earth's glaciers is a grand challenge of Earth System science. Besides being a critical parameter to model glacier evolution, knowledge of glacier volume is fundamental to quantify global sea level rise and available freshwater resources. Under current global warming Asian glaciers are losing mass, making improved glacier ice volume estimates a top priority. Direct glacier ice volume estimates are limited by difficulty in measuring the ice thickness. As a result, estimates rely on models, many of which depend on explicit physical laws but require parameters often poorly constrained. Today, the amount of satellite data is increasing at such a rate that it cannot be efficiently exploited by traditional processing pipelines. At the same time, Artificial Intelligence techniques are becoming increasingly dominant problem-solving techniques. In particular, deep learning models have recently shown the ability to surpass human accuracy in many scientific tasks. The goal of the ICENET project is to develop an innovative deep learning-based model capable of exploiting the huge amount of available satellite data to improve the current estimates of ice volumes of all High Mountain Asia glaciers. The proposed methodology makes use of state-of-the art image inpainting architectures fed with satellite-based digital elevation models (e.g. TanDEM-X), gravity and ice surface velocity data to infer subglacial topographies hence ice volumes. Modelled topographies will be constrained towards realistic solutions by using existing glacier ice thickness measurements (GlaThiDa repository). ICENET will be jointly developed by the Institute of Polar Sciences and the University of California, Irvine.
Climate Science & Modeling Earth Observation & Monitoring Computer Vision & Remote Sensing