Mitigating Climate Change Impacts on Biodiversity via Machine Learning Powered Assessment
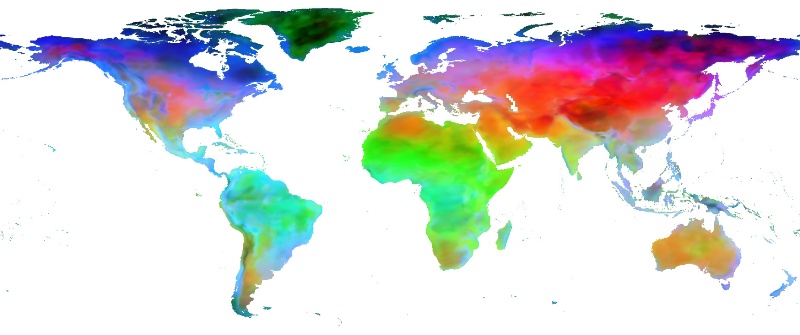
PI and co-PIs: Oisin Mac Aodha (University of Edinburgh); Scott Loarie (iNaturalist); Thomas Brooks (IUCN)
Funding amount: $145,000
Project overview: As climate change threatens biodiversity, huge gaps remain in our knowledge of where species are found and how these ranges are changing. This project seeks to infer range maps for understudied species using point observations made by citizen scientists. These range maps, built using machine learning, will be used to improve biodiversity monitoring through the iNaturalist platform by improving identification of species both by experts and by automated identification algorithms. Predicted range maps will also be integrated into official IUCN Red List assessments of previously unmapped species. This work will thus help track regional biodiversity loss and its relationship to climate change, as well as informing policies to protect vulnerable species.
Full abstract:
Click to expand
Climate change is contributing towards Earth’s sixth great extinction event. As many as one third of all species are expected to go extinct this century and with them many of the ecosystem services that sustain human life. Prioritizing scarce conservation resources to meet this crisis requires assessing threats to hundreds of thousands of species efficiently and accurately. Species distribution maps describing where species do and do not occur are critical to these assessments. The International Union for the Conservation of Nature (IUCN) has assessed the extinction risk of over 100K species and requires distribution maps accompanying assessments to identify key biodiversity areas and guide resulting conservation actions. However, generating IUCN Red List assessment distribution maps from raw species occurrence data is a labor intensive process that must be done by experts. This means that true distribution maps are generally only available for vertebrates, despite plants and insects containing many more species. We will combine species occurrences generated from the iNaturalist citizen-science platform in a machine learning model to automatically generate distributions for 50K species. By evaluating through comparison with existing IUCN Red List distribution maps, we will generate new high quality distribution maps for thousands of species for which they are as-yet-unavailable. We will learn how to better mobilize the citizen science community to collect the data necessary to scale the generation of maps required by IUCN and will make the species distribution modeling problem more accessible to the machine learning community by standardizing training datasets and evaluation procedures.
Ecosystems & Biodiversity