NeurIPS 2024 Workshop on Tackling Climate Change with Machine Learning
About
Many in the ML community wish to take action on climate change, but are unsure of the pathways through which they can have the most impact. This workshop highlights work that demonstrates that, while no silver bullet, ML can be an invaluable tool in reducing greenhouse gas emissions and in helping society adapt to the effects of climate change. Climate change is a complex problem, for which action takes many forms - from theoretical advances to deployment of new technology. Many of these actions represent high-impact opportunities for real-world change, and are simultaneously interesting academic research problems.
This workshop is part of the “Tackling Climate Change with Machine Learning” workshop series, which aims to bring together those applying ML to climate change challenges and facilitate cross-pollination between ML researchers and experts in climate-relevant fields.
Building on our past workshops on this topic, in this edition the invited talks and panels will focus on two aspects related to questioning common machine learning assumptions in the context of climate impact. Specifically, we will concentrate on two questions that are particularly timely for the machine learning community:
- Given the increasing popularity of large models (for language, vision, and other settings), what are the benefits and costs of such models in the context of climate impact?
- With the growth of specialized benchmark datasets across climate-relevant areas (including in the NeurIPS Datasets and Benchmarks Track), what makes the design of such benchmarks different from that of other ML benchmarks and how do these differences translate into impact for both ML methods and real-world impacts?
About NeurIPS 2024
This workshop will be held as part of the Conference on Neural Information Processing Systems (NeurIPS) 2024 in Vancouver, British Columbia, Canada.
About the Workshop
The main workshop took place on Dec 15, 2024. The recording and the schedule are available below, with links to papers, videos, and slides.
Schedule Full Recording
The schedule is available below, with links to papers, videos, and slides.
Time (Workshop) | Event |
---|---|
Opening Remarks
Details: (click to expand)In-person Intro, Opening Remarks |
|
Keynote Talk - Dr. Sasha Luccioni
Details: (click to expand)Title - AI Ethics Must Be Sustainable: Bridging Theory and Practice |
|
Spotlight Presentations | |
(8) "A Deep Learning Approach to the Automated Segmentation of Bird Vocalizations from Weakly Labeled Crowd-sourced Audio" | |
(73) "Multi-branch Spatio-Temporal Graph Neural Network For Efficient Ice Layer Thickness Prediction" | |
(5) "Temporal sequence transformer to advance long-term streamflow prediction" | |
(57) "Multi-scale decomposition of sea surface height snapshots using machine learning" | |
Break
Details: (click to expand)Break |
|
Keynote Talk - Dr. Esther Rolf
Details: (click to expand)Title - Where methods meet impact in ML for environmental monitoring |
|
Spotlight Presentations | |
(54) "Multi-Source Temporal Attention Network for Precipitation Nowcasting" | |
(68) "Estimating atmospheric variables from Digital Typhoon Satellite Images via Conditional Denoising Diffusion Models" | |
(60) "TAUDiff: Improving statistical downscaling for extreme-event simulation using generative diffusion models" | |
(32) "Towards turbine-location-aware multi-decadal wind power predictions with CMIP6" | |
(52) "Parakeet: Emission Factor Recommendation for Carbon Footprinting with Generative AI" | |
(70) "ATLAS: A spend classification benchmark for estimating scope 3 carbon emissions" | |
First Poster Session - Zoom Link
Details: (click to expand)There will be an in-person and a virtual poster session occuring at the same time. |
|
Lunch Break
Details: (click to expand)Break |
|
Panel Discussion – Thinking Bigger or Smaller in ML Models for Climate Impact
Details: (click to expand)Panelists: |
|
Social | |
Keynote Talk – Dr. Rebecca Hutchinson
Details: (click to expand)Title - Label Noise and Evaluation Design in Biodiversity Modeling |
|
Second Poster Session - Zoom Link
Details: (click to expand)There will be an in-person and a virtual poster session occuring at the same time. |
|
Closing Remarks + Best Paper Award
Details: (click to expand)Closing Remarks |
Speakers
Keynotes
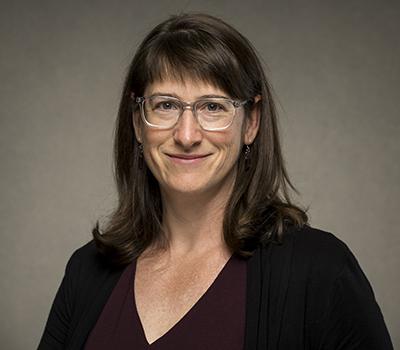
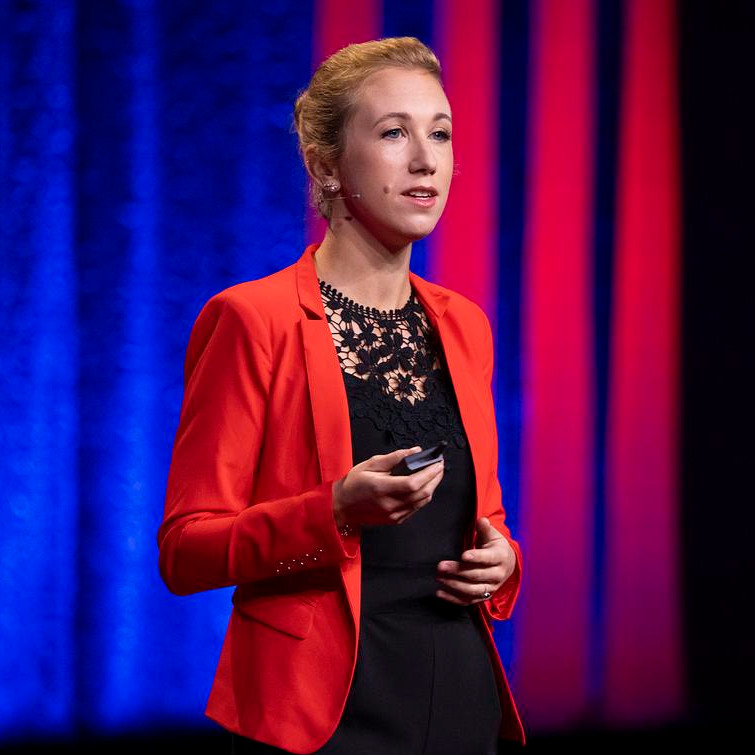
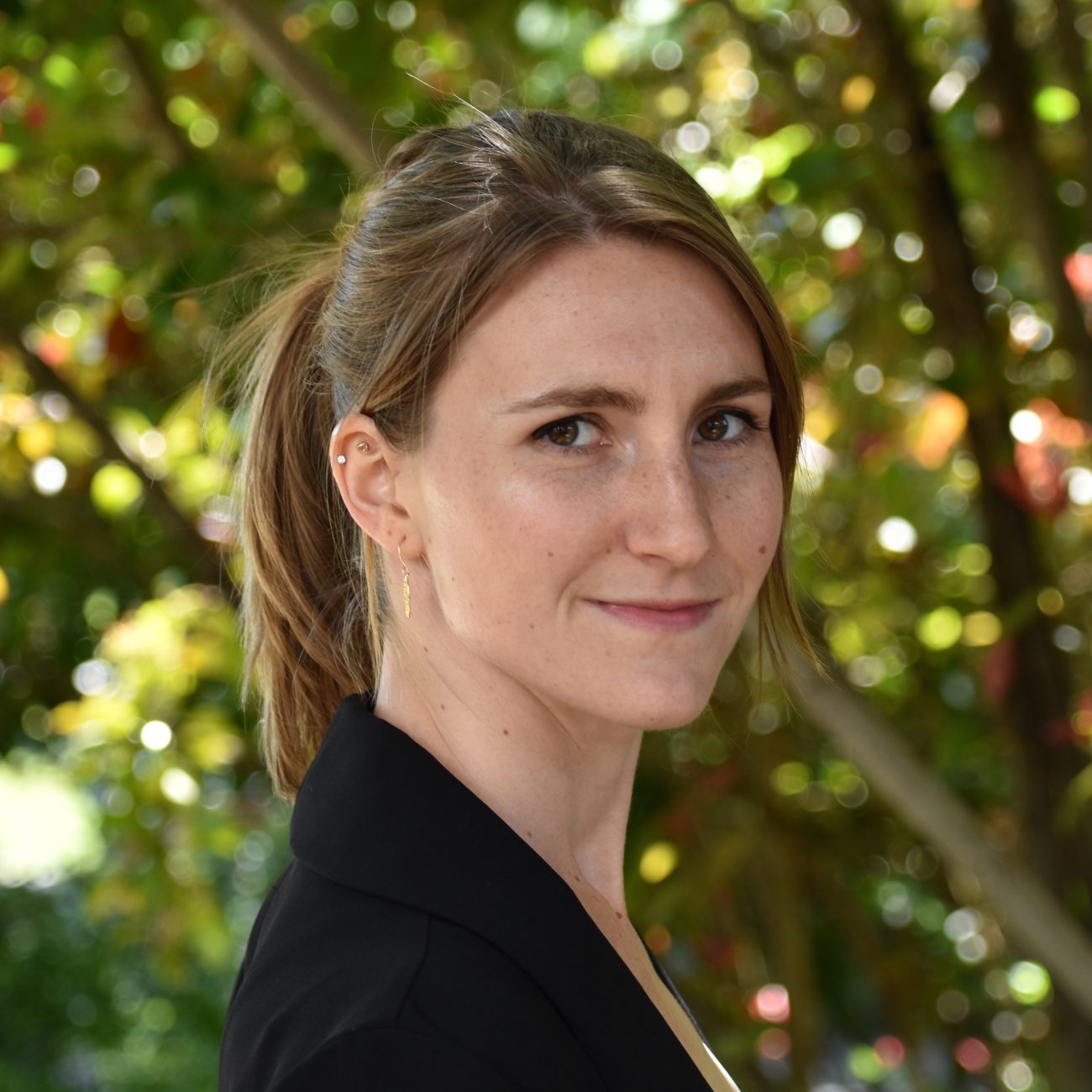
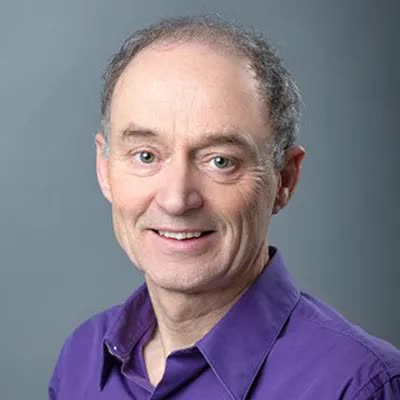
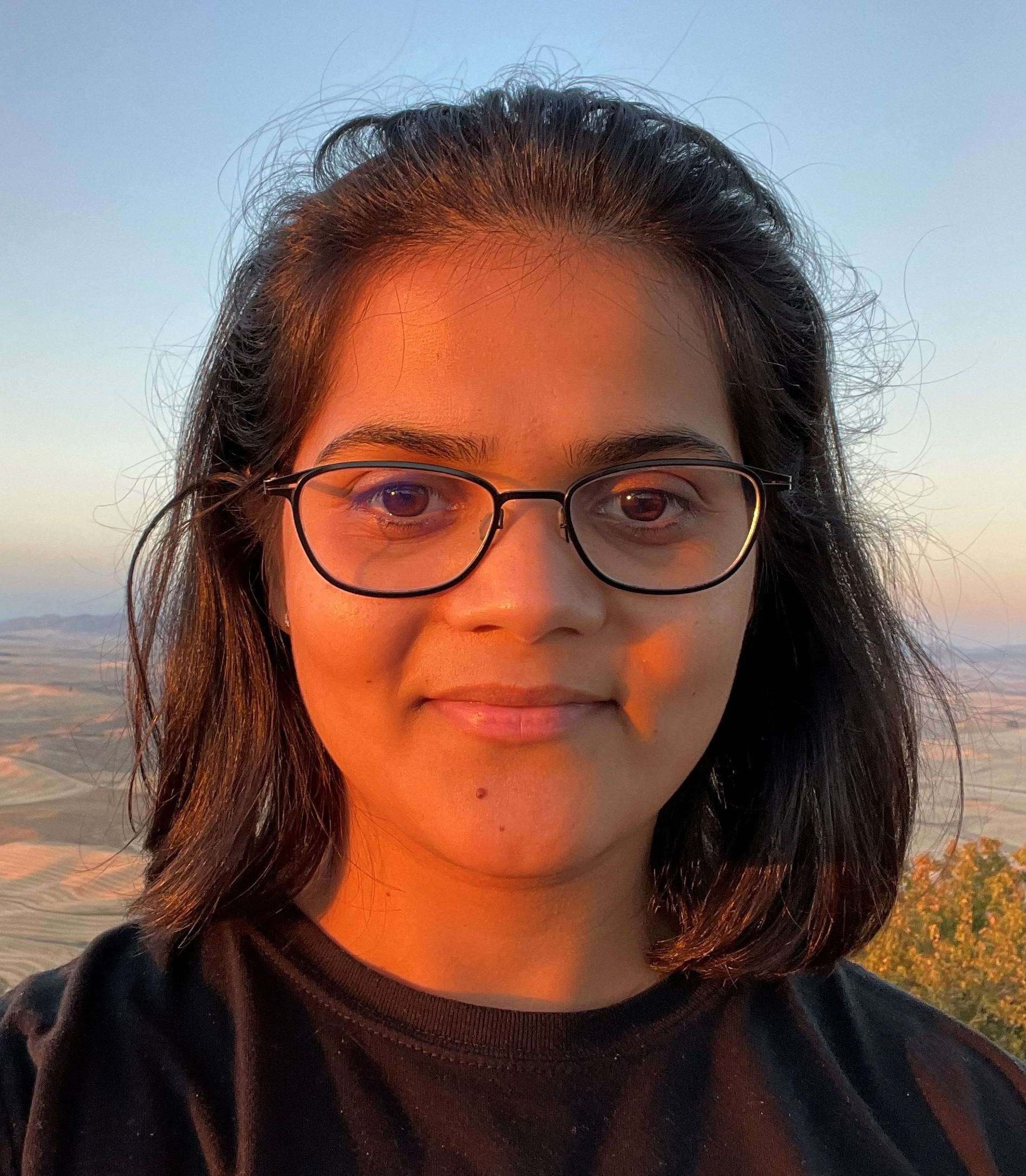
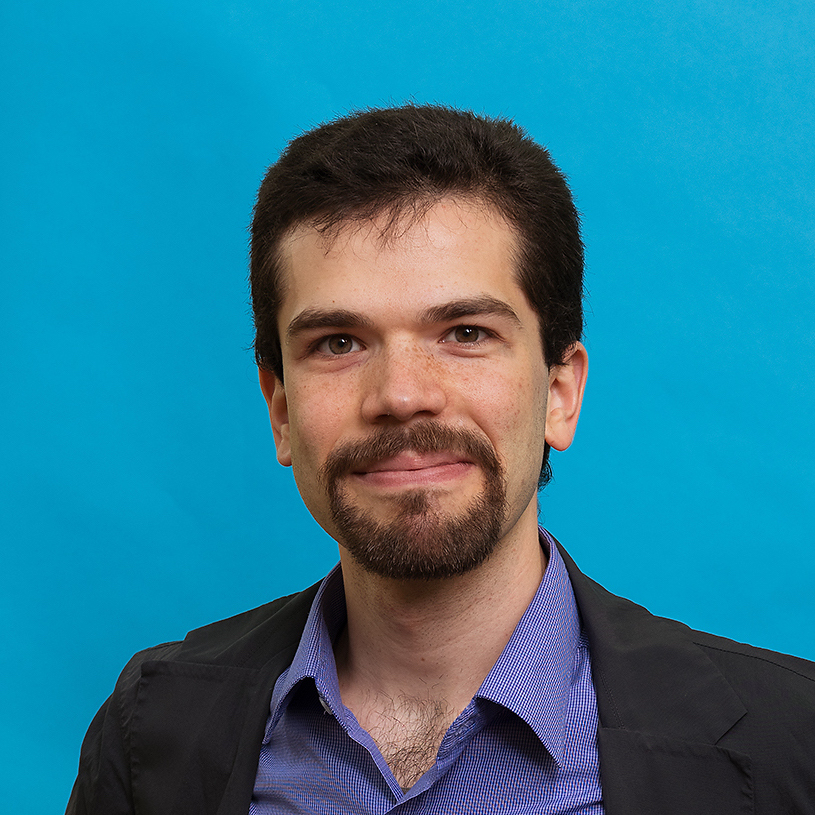
Accepted Works
Works were submitted to one of two tracks: Papers or Proposals.
Click the links below for information about each submission, including slides, videos, and papers.
Papers
Title | Authors |
---|---|
(1) Time Series Viewmakers for Robust Disruption Prediction in Nuclear Fusion | Dhruva Chayapathy (Alpharetta High School); Tavis Siebert (UC Berkeley); Lucas Spangher (Google); Cristina Rea (MIT Plasma Science and Fusion Center) |
(2) Explainable Meta Bayesian Optimization with Human Feedback for Scientific Applications like Fusion Energy | Ricardo Luna Gutierrez (Hewlett Packard Enterprise); Sahand Ghorbanpour (Hewlett Packard Enterprise); Vineet Gundecha (Hewlett Packard Enterpise); Rahman Ejaz (University of Rochester); Varchas Gopalaswamy (University of Rochester); Riccardo Betti (University of Rochester); Avisek Naug (Hewlett Packard Enterprise); Desik Rengarajan (Hewlett Packard Labs); Ashwin Ramesh Babu (Hewlett Packard Enterprise Labs); Paolo Faraboschi (Hewlett Packard Enterprise); Soumyendu Sarkar (Hewlett Packard Enterprise) |
(3) Enhancing Reinforcement Learning-Based Control of Wave Energy Converters Using Predictive Wave Modeling | Vineet Gundecha (Hewlett Packard Enterpise); Arie Paap (Carnegie Clean Energy); mathieu Cocho (Carnegie Clean Energy); Sahand Ghorbanpour (Hewlett Packard Enterprise); Alexandre Pichard (Carnegie Clean Energy); Ashwin Ramesh Babu (Hewlett Packard Enterprise Labs); Soumyendu Sarkar (Hewlett Packard Enterprise) |
(4) Satellite Sunroof: High-res Digital Surface Models and Roof Segmentation for Global Solar Mapping | Vishal Batchu (Google Research); Alex Wilson (Google); Betty Peng (Google); Carl Elkin (Google); Umangi Jain (University of Toronto); Christopher Arsdale (Google Research); Ross Goroshin (Google); Varun Gulshan (Google Research) |
(5) Temporal sequence transformer to advance long-term streamflow prediction Best ML Innovation | Ruhaan Singh (Farragut High School); Dan Lu (Oak Ridge National Laboratory); Kshitij Tayal (Oak Ridge National Laboratory) |
(6) Mapping Air Pollution Sources with Sequential Transformer Chaining: A Case Study in South Asia | Hafiz Muhammad Abubakar (Beaconhouse National University); Raahim Arbaz (Beaconhouse National University); Hasnain Ahmad (Beaconhouse National University); Mubasher Nazir (Solve Agri Pak Private Limited); Usman Nazir (Beaconhouse National University) |
(7) Icy Waters: Developing a Test-Suite to Benchmark Sea Ice Concentration Forecasting | Kiernan McGuigan (University of Waterloo); Sirisha Rambhatla (University of Waterloo); K Andrea Scott (University of Waterloo) |
(8) A Deep Learning Approach to the Automated Segmentation of Bird Vocalizations from Weakly Labeled Crowd-sourced Audio | Jacob Ayers (Engineers for Exploration at UCSD); Sean Perry (University of California San Diego); Samantha Prestrelski (UC San Diego); Tianqi Zhang (Engineers for Exploration); Ludwig von Schoenfeldt (University of California San Diego); Mugen Blue (UC Merced); Gabriel Steinberg (Demining Research Community); Mathias Tobler (San Diego Zoo Wildlife Alliance); Ian Ingram (San Diego Zoo Wildlife Alliance); Curt Schurgers (UC San Diego); Ryan Kastner (University of California San Diego) |
(9) Enabling Adoption of Regenerative Agriculture through Soil Carbon Copilots | Margaret Capetz (UCLA); Swati Sharma (Microsoft Research); Peder Olsen (Microsoft); RAFAEL PADILHA (Microsoft Research); Jessica Wolk (Microsoft); Emre Kiciman (Microsoft Research); Ranveer Chandra (Microsoft Research) |
(10) Carbon-Aware Spatio-Temporal Workload Distribution in Cloud Data Center Clusters Using Reinforcement Learning | Soumyendu Sarkar (Hewlett Packard Enterprise); Antonio Guillen-Perez (Hewlett Packard Enterprise); Vineet Gundecha (Hewlett Packard Enterpise); Avisek Naug (Hewlett Packard Enterprise); Ricardo Luna Gutierrez (Hewlett Packard Enterprise); Sajad Mousavi (Hewlett Packard Enterprise); Paolo Faraboschi (Hewlett Packard Enterprise); Cullen Bash (Hewlett Packard Enterprise) |
(11) InvestESG: A Multi-agent Reinforcement Learning Benchmark for Studying Climate Investment as a Social Dilemma | Xiaoxuan Hou (University of Washington); Jiayi Yuan (University of Washington); Natasha Jaques (University of Washington) |
(12) Exploring Vision Transformers for Early Detection of Climate Change Signals | Sungduk Yu (Intel Labs); Brian White (UNC Chapel Hill); Anahita Bhiwandiwalla (Intel Labs); Yaniv Gurwicz (Intel Labs); Musashi Hinck (Intel Corporation); Matthew Olson (Intel Labs); Raanan Rohekar (Intel Labs); Vasudev Lal (Intel Corp) |
(13) No Location Left Behind: Introducing the Fairness Assessment for Implicit Representations of Earth Data | Daniel Cai (Brown University); Randall Balestriero (Brown University) |
(14) Feasibility of Forecasting Highly Resolved Power Grid Frequency Utilizing Temporal Fusion Transformers | Hadeer El Ashhab (KIT); Benjamin Schäfer (Karlsruhe Institute of Technology); Sebastian Pütz (Karlsruhe Institute of Technology) |
(15) A Water Efficiency Dataset for African Data Centers | NOAH SHUMBA (Carnegie Mellon University Africa); Opelo Tshekiso (Carnegie Mellon University Africa); Pengfei Li (UCR); Giulia Fanti (CMU); Shaolei Ren (UC Riverside) |
(16) Using Convolutional Neural Processes to Produce High-Resolution Weather Datasets Over New Zealand | Emily O'Riordan (Bodeker Scientific) |
(17) AI-Driven Predictive Modeling of PFAS Contamination in Aquatic Ecosystems: Exploring A Geospatial Approach | Jowaria Khan (University of Michigan); David Andrews (Environmental Working Group); Kaley Beins (Environmental Working Group); Sydney Evans (Environmental Working Group); Alexa Friedman (Environmental Working Group); Elizabeth Bondi-Kelly (MIT) |
(18) End-to-End Conformal Calibration for Robust Grid-Scale Battery Storage Optimization | Christopher Yeh (California Institute of Technology); Nicolas Christianson (California Institute of Technology); Adam Wierman (California Institute of Technology); Yisong Yue (Caltech) |
(19) AtmosArena: Benchmarking Foundation Models for Atmospheric Sciences | Tung Nguyen (University of California, Los Angeles); Prateik Sinha (UCLA); Advit Deepak (University of California, Los Angeles); Karen A. McKinnon (University of California, Los Angeles); Aditya Grover (UCLA) |
(20) Harnessing AI for Wildfire Defense: An approach to Predict and Mitigate Global Fire Risk | Hassan Ashfaq (Ghulam Ishaq Khan Institute of Engineering Sciences and Technology) |
(21) Tree Species Classification using Machine Learning and 3D Tomographic SAR - a case study in Northern Europe | Jumpei Takami (United Nations Office for Outer Space Affairs); Grace Colverd (University of Cambridge); Laura Schade (Department of Energy Security and Net Zero - UKGOV); Karol Bot (INESCTEC); Joseph Gallego (Drexel University) |
(22) Towards Using Machine Learning to Generatively Simulate EV Charging in Urban Areas | Marek Miltner (Stanford University; Czech Technical University); Jakub Zíka (CTU); Daniel Vašata (Czech Technical University in Prague, Faculty of Information Technology); Artem Bryksa (CTU); Magda Friedjungová (Czech Technical University in Prague, Faculty of Information Technology); Ondřej Štogl (CTU); Ram Rajagopal (Stanford University); Oldřich Starý (CTU) |
(23) High-Resolution Domestic Energy Modelling for National Energy and Retrofit Planning | Grace Colverd (University of Cambridge); Ronita Bardhan (University of Cambridge); Jonathan Cullen (University of Cambridge) |
(24) Continuous latent representations for modeling precipitation with deep learning | Gokul Radhakrishnan (Verisk Analytics); Rahul Sundar (Verisk, India); Nishant Parashar (Verisk Analytics); Antoine Blanchard (Verisk); Daiwei Wang (Verisk Analytics); Boyko Dodov (Verisk Analytics) |
(25) Improving Power Plant CO2 Emission Estimation with Deep Learning and Satellite/Simulated Data | Dibyabha Deb (Manipal Institute of Technology); Kamal Das (IBM Research) |
(26) Advancing Heat Demand Forecasting with Attention Mechanisms: Opportunities and Challenges | Adithya Ramachandran (Pattern Recognition Lab, Friedrich Alexander University, Erlangen); Andreas Maier (Pattern Recognition Lab, FAU Erlangen-Nuremberg); Siming Bayer (Pattern Recognition Lab, Friedrich-Alexander University) |
(27) AQ-PINNs: Attention-Enhanced Quantum Physics-Informed Neural Networks for Carbon-Efficient Climate Modeling | Siddhant Dutta (SVKM's Dwarkadas J. Sanghvi College of Engineering); Nouhaila Innan (New York University Abu Dhabi); Sadok Ben Yahia (Southern Denmark University); Muhammad Shafique (New York University Abu Dhabi) |
(28) Enhancing Sustainability in Liquid-Cooled Data Centers with Reinforcement Learning Control | Avisek Naug (Hewlett Packard Enterprise); Antonio Guillen-Perez (Hewlett Packard Enterprise); Vineet Gundecha (Hewlett Packard Enterpise); Ricardo Luna Gutierrez (Hewlett Packard Enterprise); Ashwin Ramesh Babu (Hewlett Packard Enterprise Labs); Sajad Mousavi (Hewlett Packard Enterprise); Paolo Faraboschi (Hewlett Packard Enterprise); Cullen Bash (HPE); Soumyendu Sarkar (Hewlett Packard Enterprise) |
(29) Classification of Snow Depth Measurements for tracking plant phenological shifts in Alpine regions | Jan Svoboda (WSL Institute for Snow and Avalanche Research SLF); Michael Zehnder (WSL Institute for Snow and Avalanche Research SLF); Marc Ruesch (WSL Institute for Snow and Avalanche Research SLF); David Liechti (WSL Institute for Snow and Avalanche Research SLF); Corinne Jones (Swiss Data Science Center); Michele Volpi (Swiss Data Science Center, ETH Zurich); Christian Rixen (WSL Institute for Snow and Avalanche Research SLF); Jürg Schweizer (WSL Institute for Snow and Avalanche Research SLF) |
(30) Climate PAL: Climate Analysis through Conversational AI | Sonia Cromp (University of Wisconsin-Madison); Behrad Rabiei (University of California San Diego); Maxwell Elling (University of Colorado Boulder); Alexander Herron (National Aeronautics and Space Administration); Michael Hendrickson (National Aeronautics and Space Administration) |
(31) Adaptive Policy Regularization for Offline-to-Online Reinforcement Learning in HVAC Control | Hsin-Yu Liu (University of California San Diego); Bharathan Balaji (Amazon); Rajesh Gupta (UC San Diego); Dezhi Hong (Amazon) |
(32) Towards turbine-location-aware multi-decadal wind power predictions with CMIP6 | Nina Effenberger (University of Tübingen); Nicole Ludwig (University of Tübingen) |
(33) Equity-Aware Spatial-Temporal Workload Shifting for Sustainable AI Data Centers | Mohammad Islam (University of California Riverside); Shaolei Ren (UC Riverside) |
(34) Climate Impact Assessment Requires Weighting: Introducing the Weighted Climate Dataset | Marco Gortan (University of Basel); Lorenzo Testa (Carnegie Mellon University); Giorgio Fagiolo (Sant'Anna School of Advanced Studies); Francesco Lamperti (Sant'Anna School of Advanced Studies) |
(35) Meta-Learned Bayesian Optimization for Energy Yield in Inertial Confinement Fusion | Vineet Gundecha (Hewlett Packard Enterpise); Ricardo Luna Gutierrez (Hewlett Packard Enterprise); Sahand Ghorbanpour (Hewlett Packard Enterprise); Rahman Ejaz (University of Rochester); Varchas Gopalaswamy (University of Rochester); Riccardo Betti (University of Rochester); Avisek Naug (Hewlett Packard Enterprise); Paolo Faraboschi (Hewlett Packard Enterprise); Soumyendu Sarkar (Hewlett Packard Enterprise) |
(36) WildfireGPT: Tailored Large Language Model for Wildfire Analysis | Yangxinyu Xie (University of Pennsylvania); Bowen Jiang (University of Pennsylvania); Tanwi Mallick (Argonne National Laboratory); Joshua Bergerson (Argonne National Laboratory); John Hutchison (Argonne National Laboratory); Duane Verner (Argonne National Laboratory); Jordan Branham (Argonne National Laboratory); M. Ross Alexander (Argonne National Laboratory); Robert Ross (Argonne National Laboratory); Yan Feng (Argonne National Laboratory); Leslie-Anne Levy (Argonne National Laboratory); Weijie Su (University of Pennsylvania); Camillo Jose Taylor (University of Pennsylvania) |
(37) Methane SatMapper: Methane Detection from Satellite Imagery Using Hyperspectral Transformer | Satish Kumar (University of California, Santa Barbara); ASM Iftekhar (Microsoft); Bowen Zhang (University Of California, Santa Barbara); Richard Sserunjogi (Makerere University); Mehan Jayasuriya (Mozilla Technology Foundation) |
(38) A Low-Complexity Data-Driven Algorithm for Residential PV-Storage Energy Management | Mostafa Farrokhabadi (University of Calgary) |
(39) Climate Adaptation with Reinforcement Learning: Experiments with Flooding and Transportation in Copenhagen | Miguel Costa (Technical University of Denmark); Morten W. Petersen (Technical University of Denmark); Arthur Vandervoort (Technical University of Denmark); Martin Drews (Technical University of Denmark); Karyn Morrissey (Technical University of Denmark); Francisco Pereira (DTU) |
(40) Learning the Indicators of Energy Burden for Knowledge Informed Policy | Jasmine Garland (University of Colorado Boulder); Rajagopalan Balaji (University of Colorado, Boulder); Kyri Baker (University of Colorado, Boulder); Ben Livneh (University of Colorado, Boulder) |
(41) Paraformer: Parameterization of Sub-grid Scale Processes Using Transformers | Shuochen Wang (Northeastern University); Nishant Yadav (Microsoft); Auroop Ganguly (Northeastern University) |
(42) Efficient Localized Adaptation of Neural Weather Forecasting: A Case Study in the MENA Region | Muhammad Akhtar Munir (MBZUAI); Fahad Shahbaz (MBZUAI); Salman Khan (MBZUAI) |
(43) DivShift: Exploring Domain-Specific Distribution Shift in Large-Scale, Volunteer-Collected Biodiversity Datasets | Elena Sierra (Stanford University); Lauren Gillespie (Stanford University); Salim Soltani (University of Freiburg); Moisés Expósito-Alonso (University of California, Berkeley); Teja Kattenborn (University of Freiburg) |
(44) RAIN: Reinforcement Algorithms for Improving Numerical Weather and Climate Models | Pritthijit Nath (University Of Cambridge); Henry Moss (Secondmind); Emily Shuckburgh (University Of Cambridge); Mark Webb (Met Office) |
(45) Exploring Physics-Informed Neural Networks for Crop Yield Loss Forecasting | Miro Miranda (University of Kaiserslautern-Landau); Marcela Charfuelan (German Research Center for Artificial Intelligence); Andreas Dengel (DFKI GmbH) |
(46) Modeling Pollution Spread with Obstructions using Physics-Informed Neural Networks | Yash Ranjith (Westmont) |
(47) Prediction of PM2.5 concentration based on ARIMA, LSTM, and TCN models in Kigali, Rwanda | Yamlak Bogale (Carnegie Mellon University Africa); Choukouriyah Arinloye (Carnegie Mellon University Africa); Joselyne Muragijemariya (Carnegie Mellon University Africa) |
(48) Wildflower Monitoring with Expert-annotated Images and Flowering Phenology | Georgiana Manolache (Fontys University of Applied Science); Gerard Schouten (Fontys University of Applied Sciences) |
(49) Light-weight geospatial model for global deforestation attribution | Anton Raichuk (Google); Michelle Sims (WRI); Radost Stanimirova (WRI); Maxim Neumann (Google) |
(50) HVAC-DPT: A Decision Pretrained Transformer for HVAC Control | Anaïs Berkes (University of Cambridge) |
(51) Regional Ocean Forecasting with Hierarchical Graph Neural Networks | Daniel Holmberg (University of Helsinki); Emanuela Clementi (CMCC Foundation); Teemu Roos (University of Helsinki) |
(52) Parakeet: Emission Factor Recommendation for Carbon Footprinting with Generative AI | Bharathan Balaji (Amazon); Nina Domingo (Amazon); Abu Zaher Faridee (Amazon); Venkata Sai Gargeya Vunnava (amazon); Anran Wang (Amazon); Fahimeh Ebrahimi Meymand (Amazon); Kellen Axten (Amazon); Aravind Srinivasan (Amazon); Qingshi Tu (University of British Columbia); Harsh Gupta (Amazon); Shikha Gupta (Amazon); Soma Ramalingam (Amazon); Jeremie Hakian (Amazon); Jared Kramer (Amazon) |
(53) Towards CLEAN: Cycle Learning Energy-Emission Assessment Network | Yanming Guo (University of Sydney); Jin Ma (University of Sydney); Kevin Credit (Maynooth University); Qian Xiao (Maynooth University) |
(54) Multi-Source Temporal Attention Network for Precipitation Nowcasting Best Pathway to Impact | Rafael Pablos Sarabia (Aarhus University & Cordulus); Joachim Nyborg (Cordulus); Morten Birk (Cordulus); Jeppe Liborius Sjørup (Cordulus); Anders Lillevang Vesterholt (Cordulus); Ira Assent (Aarhus University) |
(55) Critical misalignments between climate action and sustainable development goals revealed | Francesca Larosa (Royal Institute for Technology); Sergio Hoyas (Universitat Politècnica de València); Fermin Mallor Franco (Royal Institute of Technology); J. Alberto Conejero (Universitat Politècnica de València); Javier García-Martinez (University of Alicante); Francesco Fuso Nerini (Royal Institute of Technology); Ricardo Vinuesa (KTH Royal Institute of Technology) |
(56) Clustering-Based Framework for Assessing Transportation Resilience to Flood Events | Matheus Pedra (TECNUN); Leire Labaka (TECNUN); Josune Hernantes (TECNUN) |
(57) Multi-scale decomposition of sea surface height snapshots using machine learning | Yue Wang (Columbia University); Jingwen Lyu (Columbia University); Chris Pedersen (NYU); Spencer Jones (Texas A&M University); Dhruv Balwada (Columbia University) |
(58) Safe Reinforcement Learning for Remote Microgrid Optimization with Industrial Constraints | Hadi Nekoei (Mila); Alexandre Blondin Massé (Hydro-Quebec); Rachid Hassani (Hydro-quebec); Sarath Chandar (Mila / École Polytechnique de Montréal); Vincent Mai (Hydro-Québec) |
(59) CanadaFire2023: Burned Area Mapping Datasets and Benchmarks for Canadian Wildfires in 2023 | Zilong Zhong (McMaster University); Alemu Gonsamo (McMaster University) |
(60) TAUDiff: Improving statistical downscaling for extreme-event simulation using generative diffusion models | Rahul Sundar (Verisk, India); Nishant Parashar (Verisk); Antoine Blanchard (Verisk); Boyko Dodov (Verisk) |
(61) Emulating the Global Change Analysis Model with Deep Learning | Andrew Holmes (Western Washington University); Matt Jensen (Pacific Northwest National Laboratory); Sarah Coffland (Western Washington University); Hidemi Mitani-Shen (Western Washington University); Logan Sizemore (Western Washington University); Seth Bassetti (Utah State University); Brenna Nieva (Western Washington University); Claudia Tebaldi (Joint Global Change Research Institute); Abigail Snyder (Joint Global Change Research Institute); Brian Hutchinson (Western Washington University) |
(62) Sliced-Wasserstein-based Anomaly Detection and Open Dataset for Localized Critical Peak Rebates | Julien Pallage (Polytechnique Montréal, Mila, GERAD); Bertrand Scherrer (Hydro-Québec); Salma Naccache (Hydro-Québec); Christophe Bélanger (Hydro-Québec); Antoine Lesage-Landry (Polytechnique Montréal & GERAD) |
(63) Scalable and interpretable deforestation detection in the Amazon rainforest | Rodrigo Schuller (IMPA); Francisco Ganacim (IMPA); Paulo Orenstein (IMPA) |
(64) Operational Wind Speed Forecasts for Chile's Electric Grid Using A Hybrid Machine Learning Model | Dhruv Suri (Stanford University); Praneet Dutta (Google); Flora Xue (Google DeepMind); Ines Azevedo (Stanford University); Ravi Jain (X (formerly Google X)) |
(65) Predicting NOx emissions in Biochar Production Plants using Machine Learning | Marius Köppel (Johannes Gutenberg University Mainz); Tobias Schweitzer (AIRA Holding GmbH); Jochen Weber (None); Erdem Yilmaz (PYREG GmbH); Niklas Witzig (Johannes Gutenberg University Mainz); Tim Klausmann (Johannes Gutenberg University Mainz); Mattia Cerrato (JGU Mainz); Juan Chimbo (ARTi); Bernardo del Campo (ARTi); Lissete Davila (ARTi); David Barreno (ARTi) |
(66) Spatio-Temporal Machine Learning Models for Emulation of Global Atmospheric Composition | Mohammad Erfani (Columbia University); Kara Lamb (Columbia University); Susanne Bauer (NASA Goddard Institute for Space Studies); Kostas Tsigaridis (Columbia University); Marcus van Lier-Walqui (Columbia University); Gavin Schmidt (NASA Goddard Institute for Space Studies) |
(67) Probabilistic representation learning of subseasonal to seasonal ocean dynamics | Jannik Thuemmel (University of Tuebingen); Jakob Schlör (ECMWF); Florian Ebmeier (University of Tuebingen); Bedartha Goswami (University of Tübingen) |
(68) Estimating atmospheric variables from Digital Typhoon Satellite Images via Conditional Denoising Diffusion Models | Zhangyue Ling (Imperial College London); Pritthijit Nath (University Of Cambridge); Cesar Quilodran-Casas (Imperial College London) |
(69) RL for Mitigating Cascading Failures: Targeted Exploration via Sensitivity Factors | Anmol Dwivedi (RPI); Ali Tajer (RPI); Santiago Paternain (Rensselaer Polytechnic Institute); Nurali Virani (GE Research) |
(70) ATLAS: A spend classification benchmark for estimating scope 3 carbon emissions | Andrew Dumit (Watershed Technology, Inc.); Krishna Rao (Watershed Technology, Inc.); Travis Kwee (Watershed Technology, Inc.); Varsha Gopalakrishnan (Watershed Technology Inc.); Katherine Tsai (Watershed Technology, Inc.); Sangwon Suh (Watershed Technology, Inc.) |
(71) Hierarchical Classification for Automated Image Annotation of Coral Reef Benthic Structures | Celia Blondin (IRD); Joris Guerin (IRD, Univ. Montpellier); Laure Berti-Equille (IRD); Guilherme Ortigara Longo (Universidade Federal do Rio Grande do Norte); Kelly Inagaki (Universidade Federal do Rio Grande do Norte) |
(72) Optimizing NMR Spectroscopy Pulse Sequencing with Reinforcement Learning for Soil Atomic Abundance | Rohan Shenoy (UC Berkeley); Hans Gaensbauer (MIT); Elsa Olivetti (MIT); Evan Coleman (MIT) |
(73) Multi-branch Spatio-Temporal Graph Neural Network For Efficient Ice Layer Thickness Prediction Overall Best Paper | Zesheng Liu (Lehigh University); Maryam Rahnemoonfar (Lehigh University) |
(74) Resolution-Agnostic Transformer-based Climate Downscaling | Declan Curran (UNSW); Hira Saleem (UNSW); Sanaa Hobeichi (The University of New South Wales); Flora Salim (University of New South Wales) |
(75) Scalable Satellite Imagery Analysis: A Cascade Framework for Sparse Target Detection | Arvind Manivannan (University of Washington); Tarun Narayanan Venkatachalam (Allen Institute for AI); Yanlin Huang (University of Washington); Favyen Bastani (Allen Institute for AI) |
(76) Estimating Greenland Ice Sheet Dynamics Using Neural Operators | Maryam Rahnemoonfar (Lehigh University); Heling Wang (Lehigh University) |
(77) Enhanced Detection of Human-Driven Forest Alterations using Echo State Networks | Tomás Couso (PUC); Paula Aguirre (PUC); Rodrigo Carrasco (PUC); Javier Lopatin (UAI) |
Proposals
Title | Authors |
---|---|
(78) Optimizing Carbon Emissions and Cost Reductions for Household Energy Demand Using Machine Learning | Shivani Chotalia (Clean AI Initiative); Kyungmin Lee (University of Delaware); Soazig Kaam (N/A); Victor Hutse (Radix); David Quispe (University of Toronto) |
(79) Machine Learning Models for Predicting Solar Power Potential and Energy Efficiency in some underserved localities of the Congo Basin Region | Jean de Dieu NGUIMFACK NDONGMO (The University of Bamenda); Adelaide Nicole KENGNOU TELEM (University of Buea); Reeves MELI FOKENG (The University of Bamenda) |
(80) A Hybrid Machine Learning Model For Ship Speed ThroughWater: Solve And Predict | Zakarya ELMIMOUNI (ENSAE); Ayoub Atanane (UQAR); Loubna Benabbou (UQAR) |
(81) Enhanced Cruise Control for Reducing Automobile Environmental Impact | Shubhankar Gahlot (University of Canterbury NZ) |
(82) Exploring Climate Awareness and Anxiety in Teens: An Expert-Driven AI Perspective | Sruthi Viswanathan (University of Oxford and The Spaceship Academy); Omar Mohammed (Independant Researcher); Craig Vezina (The Spaceship Academy); Mia Doces (Commitee For Children) |
(83) Generating Climate Dataset in a Data-scarce Region of Choke Mountain Watersheds in Ethiopia Using Machine Learning Techniques | Sintayehu Abebe (Debre Markos University); Kassahun Tadesse (Debre Markos University); Mulu Kerebih (Debre Markos University); Bekalu Asres (Debre Markos University); Bewketu Mulu (Debre Markos University); Varsha Gopalakrishnan (Self) |
(84) Mamba MethaneMapper: State Space Model for Methane Detection from Hyperspectral Imagery | Satish Kumar (University of California, Santa Barbara); ASM Iftekhar (Microsoft); Kaikai Liu (University of California, Santa Barbara); Bowen Zhang (University Of California, Santa Barbara); Mehan Jayasuriya (Mozilla) |
(85) Unlocking the Potential of Green Virtual Bidding Strategies : A Pathway to a Low-Carbon Electricity Market | Aya Laajil (Centrale Supelec); Loubna Benabou (UQAR); Frédérique M. Gagnon (Videns Analytics); Laurent Barcelo (Videns Analytics); Ghait Boukachab (Videns Analytics & Mila) |
(86) Cross-Border Electricity Price Forecasting with Deep Learning | Hadeer El Ashhab (KIT); Benjamin Schäfer (Karlsruhe Institute of Technology) |
(87) Making Climate AI Systems Past and Future Aware to Better Evaluate Climate Change Policies | Riya . (IIT Roorkee); Sudhakar Singh (Nvidia) |
(88) A Novel Approach to Assessing the Efficacy of Policy-driven Urban Energy Demand Reduction via Proximal Infrared Remote Sensing | Kyungmin Lee (University of Delaware); Gregory Dobler (University of Delaware) |
(89) Composing Open-domain Vision with RAG for Ocean Monitoring and Conservation | Sepand Dyanatkar (OnDeck Fisheries AI); Angran Li (OnDeck Fisheries AI); Alexander Dungate (OnDeck Fisheries AI) |
(90) How are companies reducing emissions? An LLM-based approach to creating a carbon emissions reduction levers library at scale | Varsha Gopalakrishnan (Watershed Technology Inc.); Shaena Ulissi (Watershed); Andrew Dumit (Watershed Technology, Inc.); Krishna Rao (Watershed Technology Inc.); Katherine Tsai (Watershed Technology Inc.); Sangwon Suh (Watershed Technology Inc.) |
(91) Enhanced PINNs for high-order power grid dynamics | Vineet Jagadeesan Nair (MIT) |
(92) Multimodal AI framework for predicting candidate high temperature superconductors | Nidhish Sagar (Massachusetts Institute of Technology); Eslam G. Al-Sakkari (Polytechnique Montréal); Ahmed Ragab (Polytechnique Montréal) |
(93) Seeing Inside Buildings: Leveraging Generative AI and Multimodal Data to Automate Building Material Audits | Nikita Klimenko (MIT); James Stoddart (Autodesk, Inc.); Lorenzo Villaggi (Autodesk, Inc.); Dale Zhao (Autodesk, Inc.) |
(94) Flood Prediction in Kenya - Leveraging Pre-Trained Models to Generate More Validation Data in Sparse Observation Settings | Alim Karimi (Self / Purdue University); David Quispe (University of Toronto); Hammed Akande (Concordia University); Nicole Mong'are (Athi Water Works Development Agency); Valerie Brosnan (Mitga Solutions); Asbina Baral (Ministry of Education, Science and Technology) |
(95) Towards more efficient agricultural practices via transformer-based crop type classification | Isabella Smythe (Columbia University); Eduardo Ulises Moya (Gobierno de Jalisco); Michael Smith (Aspia Space); Yazid Mikail (Climate Change AI); Daisy Ondwari (Kabarak University) |
(96) A Multimodal Causal Framework for Large-Scale Ecosystem Valuation: Application to Wetland Benefits for Flood Mitigation | Hannah Druckenmiller (Caltech); Georgia Gkioxari (Caltech); Connor Jerzak (University of Texas at Austin); SayedMorteza Malaekeh (University of Texas at Austin) |
(97) Stubble (Crop Residue) Burning Detection Through Satellite Images Using Geospatial Foundation Model: A Case Study in Punjab, India | Rajiv Ranjan (Plaksha University); Ying-Jung Chen (Georgia Institute of Technology); Shashank Tamaskar (Plaksha University); Anupam Sobti (IIT Delhi) |
(98) Leveraging Artificial Intelligence for Real-Time Carbon Emission Management and Climate Change Resilience Planning in Kenya | Alukwe Jones (Kabarak University); Theophilus Owiti (Kabarak University); Mercy Gachoka (Kabarak Univeristy); Peter Rogendo (Kabarak University) |
(99) DeepMyco - Dataset Generation for Dye Mycoremediation | Danika Gupta (The Harker Upper School) |
(100) Large language model co-pilot for transparent and trusted life cycle assessment comparisons | Nathan Preuss (Cornell University); Fengqi You (Cornell University) |
Call for Submissions
We invite submissions of short papers using machine learning to address problems in climate mitigation, adaptation, or modeling, including but not limited to the following topics:
- Agriculture and food
- Behavioural and social science
- Buildings
- Carbon capture and sequestration
- Cities and urban planning
- Climate finance and economics
- Climate justice
- Climate science and climate modeling
- Disaster management and relief
- Earth observations and monitoring
- Earth science
- Ecosystems and biodiversity
- Extreme weather
- Forestry and other land use
- Health
- Heavy industry and manufacturing
- Local and indigenous knowledge systems
- Materials science and discovery
- Oceans and marine systems
- Power and energy systems
- Public policy
- Societal adaptation and resilience
- Supply chains
- Transportation
All machine learning techniques are welcome, from kernel methods to deep learning. Each submission should make clear why the application has (or could have) a pathway to positive impacts regarding climate change. We highly encourage submissions which make their data publicly available. Accepted submissions will be invited to give poster presentations, of which some will be selected for spotlight talks.
The workshop does not publish proceedings, and submissions are non-archival. Submission to this workshop does not preclude future publication. Previously published work may be submitted under certain circumstances (see the FAQ).
All papers and proposals submissions must be through the submission website. Submissions will be reviewed double-blind; do your best to anonymize your submission, and do not include identifying information for authors in the PDF. Authors are required to use the workshop style template (based on the NeurIPS style files), available for LaTeX and docx format.
Please see the Tips for Submissions and FAQ, and contact climatechangeai.neurips2024@gmail.com with questions.
Submission Tracks
There are three tracks for submissions: (i) Papers, (ii) Proposals. Submissions are limited to 4 pages for the Papers track, and 3 pages for the Proposals track, in PDF format (see examples from past workshops). References do not count towards this total. Supplementary appendices are allowed but will be read at the discretion of the reviewers. All submissions must explain why the proposed work has (or could have) positive impacts regarding climate change.
PAPERS Track
Work that is in progress, published, and/or deployed.
Submissions for the Papers track should describe projects relevant to climate change that involve machine learning. These may include (but are not limited to) academic research; deployed results from startups, industry, public institutions, etc.; and climate-relevant datasets.
Submissions should provide experimental or theoretical validation of the method presented, as well as specifying what gap the method fills. Authors should clearly illustrate a pathway to climate impact, i.e., identify the way in which this work fits into broader efforts to address climate change. Algorithms need not be novel from a machine learning perspective if they are applied in a novel setting. Details of methodology need not be revealed if they are proprietary, though transparency is highly encouraged.
Submissions creating novel datasets are welcomed. Datasets should be designed to permit machine learning research (e.g. formatted with clear benchmarks for evaluation). In this case, baseline experimental results on the dataset are preferred, but not required.
PROPOSALS Track
Early-stage work and detailed descriptions of ideas for future work.
Submissions for the Proposals track should describe detailed ideas for how machine learning can be used to solve climate-relevant problems. While less constrained than the Papers track, Proposals will be subject to a very high standard of review. Ideas should be justified as extensively as possible, including motivation for why the problem being solved is important in tackling climate change, discussion of why current methods are inadequate, explanation of the proposed method, and discussion of the pathway to climate impact. Preliminary results are optional.
Tips for Submissions
- For examples of typical formatting and content, see submissions from our previous workshops.
- Be explicit: Describe how your proposed approach addresses climate change, demonstrating an understanding of the application area.
- Frame your work: The specific problem and/or data proposed should be contextualized in terms of prior work.
- Address the impact: Describe the practical implications of your method in addressing the problem you identify, as well as any relevant societal impacts or potential side-effects. We recommend reading our further guidelines on this aspect here.
- Explain the ML: Readers may not be familiar with the exact techniques you are using or may desire further detail.
- Justify the ML: Describe why the ML method involved is needed, and why it is a good match for the problem.
- Avoid jargon: Jargon is sometimes unavoidable but should be minimized. Ideal submissions will be accessible both to an ML audience and to experts in other relevant fields, without the need for field-specific knowledge. Feel free to direct readers to accessible overviews or review articles for background, where it is impossible to include context directly.
Addressing Impact
Tackling climate change requires translating ideas into action. The guidelines below will help you clearly present the importance of your work to a broad audience, hopefully including relevant decision-makers in industry, government, nonprofits, and other areas.
- Illustrate the link: Many types of work, from highly theoretical to deeply applied, can have clear pathways to climate impact. Some links may be direct, such as improving solar forecasting to increase utilization within existing electric grids. Others may take several steps to explain, such as improving computer vision techniques for classifying clouds, which could help climate scientists seeking to understand fundamental climate dynamics.
- Consider your target audience: Try to convey with relative specificity why and to whom solving the problem at hand will be useful. If studying extreme weather prediction, consider how you would communicate your key findings to a government disaster response agency. If analyzing a supply chain optimization pilot program, what are the main takeaways for industries who might adopt this technology? To ensure your work will be impactful, where possible we recommend co-developing projects with relevant stakeholders or reaching out to them early in the process for feedback. We encourage you to use this opportunity to do so!
- Outline key metrics: Quantitative or qualitative assessments of how well your results (or for proposals, anticipated results) compare to existing methods are encouraged. Try to give a sense of the importance of your problem and your findings. We encourage you to convey why the particular metrics you choose are relevant from a climate change perspective. For instance, if you are evaluating your machine learning model on the basis of accuracy, how does improved accuracy on a machine learning model translate to climate impact, and why is accuracy the best metric to use in this context?
- Be clear and concise: The discussion of impact does not need to be lengthy, just clear.
- Convey the big picture: Ultimately, the goal of Climate Change AI is to “empower work that meaningfully addresses the climate crisis.” Try to make sure that from the beginning, you contextualize your method and its impacts in terms of this objective.
Organizers
Tejasri Nampally (Indian Institute of Technology Hyderabad)
Amrita Gupta (Microsoft)
Diego Kiedanski (Tryolabs)
Yazid Salahudeen Mikail (Ahmadu Bello University)
Arthur Ouaknine (McGill, Mila)
Bistra Dilkina (University of Southern California)
Yoshua Bengio (Mila, UdeM)
Mentorship Program
We are hosting a mentorship program to facilitate exchange between potential workshop submitters and experts working in topic areas relevant to the workshop. The goal of this program is to foster cross-disciplinary collaborations and ultimately increase the quality and potential impact of submitted work.
Expectations
Mentors are expected to guide mentees during the CCAI mentorship program as they prepare submissions for this workshop.
Examples of mentor-mentee interactions may include:
- In-depth discussion of relevant related work in the area of the Paper or Proposal, to ensure submissions are well-framed and contextualized in terms of prior work.
- Giving feedback on the writing or presentation of a Paper or Proposal to bring it to the right level of maturity for submission.
Mentees are expected to initiate contact with their assigned mentor and put in the work and effort necessary to prepare a Paper or Proposal submission by August 29th, 2024, AoE.
We suggest that after the mentor-mentee matching is made, a first (physical or digital) meeting should take place within the first week (Aug 12-16) to discuss the Paper or Proposal and set expectations for the mentorship period. Subsequent interactions can take place either through meetings or via email discussions, following the expectations set during the initial meeting, culminating in a final version of a Paper or Proposal submitted via the CMT portal by August 29th.
Mentors and mentees must abide by the Climate Change AI Code of Conduct: https://www.climatechange.ai/code_of_conduct.
Informational Webinar
We have conducted two informational webinars answering questions about the mentorship program and how to prepare a successful submission for the workshop, for previous instances of the workshop. The video recordings can be found here.
Sponsors
Gold Sponsor
Bronze Sponsor
Frequently Asked Questions
Mentorship Program FAQ
Q: Are mentors allowed to be authors on the paper for which they provided mentorship?
A: Yes, mentors can be co-authors but not reviewers.
Q: What happens if the mentor/mentee does not fulfill their duties, or if major issues come up?
A: Please email us at climatechangeai.neurips2024@gmail.com and we will do our best to help resolve the situation. Potential breaches of the Code of Conduct will be responded to promptly as detailed therein.
Q: What happens if I apply to be a mentee but do not get paired with a mentor?
A: While we will do our best, we cannot guarantee pairings for everyone. Even if you do not get paired with a mentor, we encourage you to submit a Paper or Proposal to the workshop, and our reviewers will provide you with guidance and feedback on how to improve it.
Q: What happens if my submission does not get accepted to the workshop?
A: While the mentorship program is meant to give early-career researchers and students the opportunity to improve the quality of their work, sometimes submissions will need further polishing and elaboration before being ready for presentation at a CCAI workshop. If this is the case, we invite you to take into account the comments made by the reviewers and to resubmit again to a subsequent CCAI workshop.
Q: I cannot guarantee that I can commit at least 4 hours to the program over the time period. Should I still apply as a mentor?
A: No. While the 4 hour time commitment is a suggestion, we do believe that it is necessary to ensure that all mentees receive the help and guidance they need.
Q: I do not have a background in machine learning; can I still apply to be a mentor/mentee?
A: Yes! We welcome applications from domains that are complementary to machine learning to solve the problems that we are targeting.
Q: What happens if my mentor/mentee wants to continue meeting after the workshop?
A: We welcome and encourage continued interactions after the official mentorship period. That said, neither the mentor nor the mentee should feel obligated to maintain contact.
Submission FAQ
Q: How can I keep up to date on this kind of stuff?
A: Sign up for our mailing list!
Q: I’m not in machine learning. Can I still submit?
A: Yes, absolutely! We welcome submissions from many fields. Do bear in mind, however, that the majority of attendees of the workshop will have a machine learning background; therefore, other fields should be introduced sufficiently to provide context for the work.
Q: What if my submission is accepted but I can’t attend the workshop?
A: You may ask someone else to present your work in your stead. In exceptional circumstances (e.g., visa issues), on a case-by-case basis, people will be able to send in a recording instead of being there in person.
Q: It’s hard for me to fit my submission on 3 or 4 pages. What should I do?
A: Feel free to include appendices with additional material (these should be part of the same PDF file as the main submission). Do not, however, put essential material in an appendix, as it will be read at the discretion of the reviewers.
Q: Can I send submissions directly by email?
A: No, please use the CMT website to make submissions.
Q: The submission website is asking for my name. Is this a problem for anonymization?
A: You should fill out your name and other info when asked on the submission website; CMT will keep your submission anonymous to reviewers.
Q: Do submissions for the Proposals track need to have experimental validation?
A: No, although some initial experiments or citation of published results would strengthen your submission.
Q: The submission website never sent me a confirmation email. Is this a problem?
A: No, the CMT system does not send automatic confirmation emails after a submission, though the submission should show up on the CMT page once submitted. If in any doubt regarding the submission process, please contact the organizers. Also please avoid making multiple submissions of the same article to CMT.
Q: Can I submit previously published work to this workshop?
A: Yes, though under limited circumstances. In particular, work that has previously been published at non-machine learning venues may be eligible for submission; however, work that has been published in conferences on machine learning or related fields is likely not eligible. If your work was previously accepted to a Climate Change AI workshop, this work should have changed or matured substantively to be eligible for resubmission. Please contact climatechangeai.neurips2024@gmail.com with any questions.
Q: Can I submit work to this workshop if I am also submitting to another NeurIPS 2024 workshop?
A: Yes. We cannot, however, guarantee that you will not be expected to present the material at a time that conflicts with the other workshop.