The In-Person Climate Change AI Summer School: 2024 Recap
Read about the 2024 edition of CCAI’s in-person program and sign up to be notified when applications for the next iteration of the program open!
The climate change and AI space is growing rapidly, requiring democratization of expertise on these topics as well as the development of leaders who are able to shape the space responsibly. In August, we brought together 34 future leaders to the Mila - Quebec AI Institute in Montreal to spend a week together expanding their skills in the field. During this time, participants engaged with training sessions on project development skills, touching on topics such as pathways to impact and responsible AI. Their learnings were immediately put to use: under the guidance of expert mentors, participants worked together in teams to develop projects using AI to solve various climate related challenges. The summer school also focused heavily on building lasting relationships among participants through social and networking events. Keep reading for the 2024 Summer School highlights, a closer look into each team’s project, and how to get involved with the next edition of the program.
A Diverse Cohort
CCAI’s In-Person Summer School is a project-based program geared towards individuals with background in AI and/or a climate change-related field, across many career stages. We received over 850 applications for this year’s program and were excited to be able to bring 34 of those applicants to Montreal. This year’s cohort was curated to span across careers and areas of knowledge: participants ranged from activists, to researchers, to government officials, with expertise in topics such as hydrology, policy-making and data science, and came from over twenty five countries.
“Throughout the summer school, I had the opportunity to connect with a passionate and talented community of individuals dedicated to leveraging AI for climate action. The relationships forged and insights gained will undoubtedly lead to exciting collaborations and collective impact in the future. Overall, the CCAI Summer School has been a catalyst for my growth as a climate advocate and has equipped me with the knowledge and network to make meaningful contributions at the intersection of climate and AI.”
– Nabila Putri Salsabila, In-Person Summer School Participant
Training Sessions and Social Events
The Summer School was a fantastic opportunity for participants to gain hands-on experience working with an interdisciplinary team on an AI-for-climate project, supported by workshops and discussion sessions that enabled participants to produce more impactful projects, become thought leaders and connect as a cohort. Our instructors delivered training sessions on skills that are important for work at the intersection of climate change and machine learning: pathway to impact, responsible AI, working effectively in interdisciplinary teams, and effective communication. The participants took the learnings from these sessions and applied them to inform the responsible development of their projects and create compelling pitches. For example, the sessions led to one team researching the uses of AI to enhance satellite imagery to explore the potential ethical drawbacks of their proposed solution.
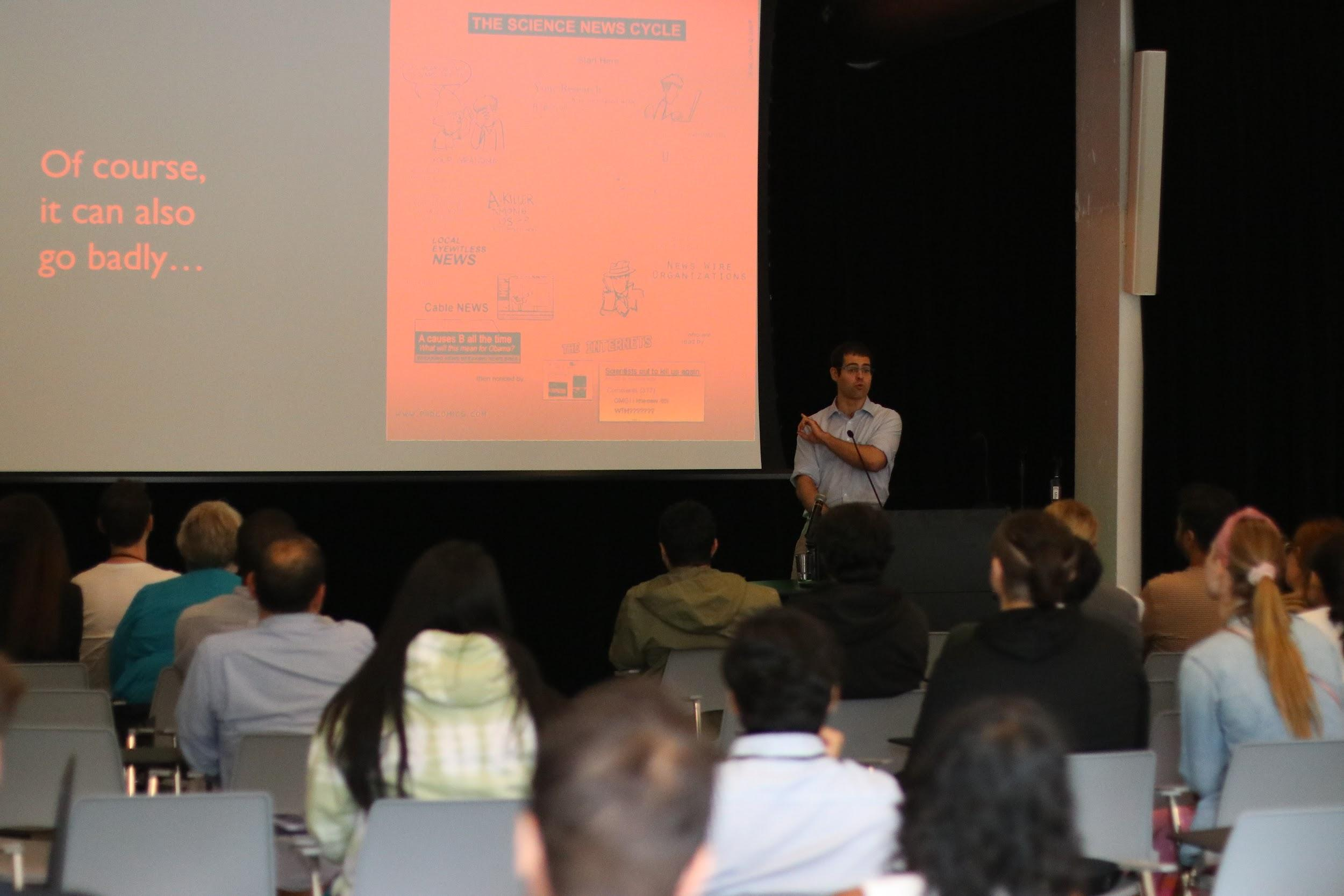
The summer school was not only work – there was fun too. For instance, during a 5à7 at Mila, participants got to know each other better and networked with some members of the Mila ecosystem. Participants also got to visit Montreal’s TimeOut Market, and explored the city together.
Project Development
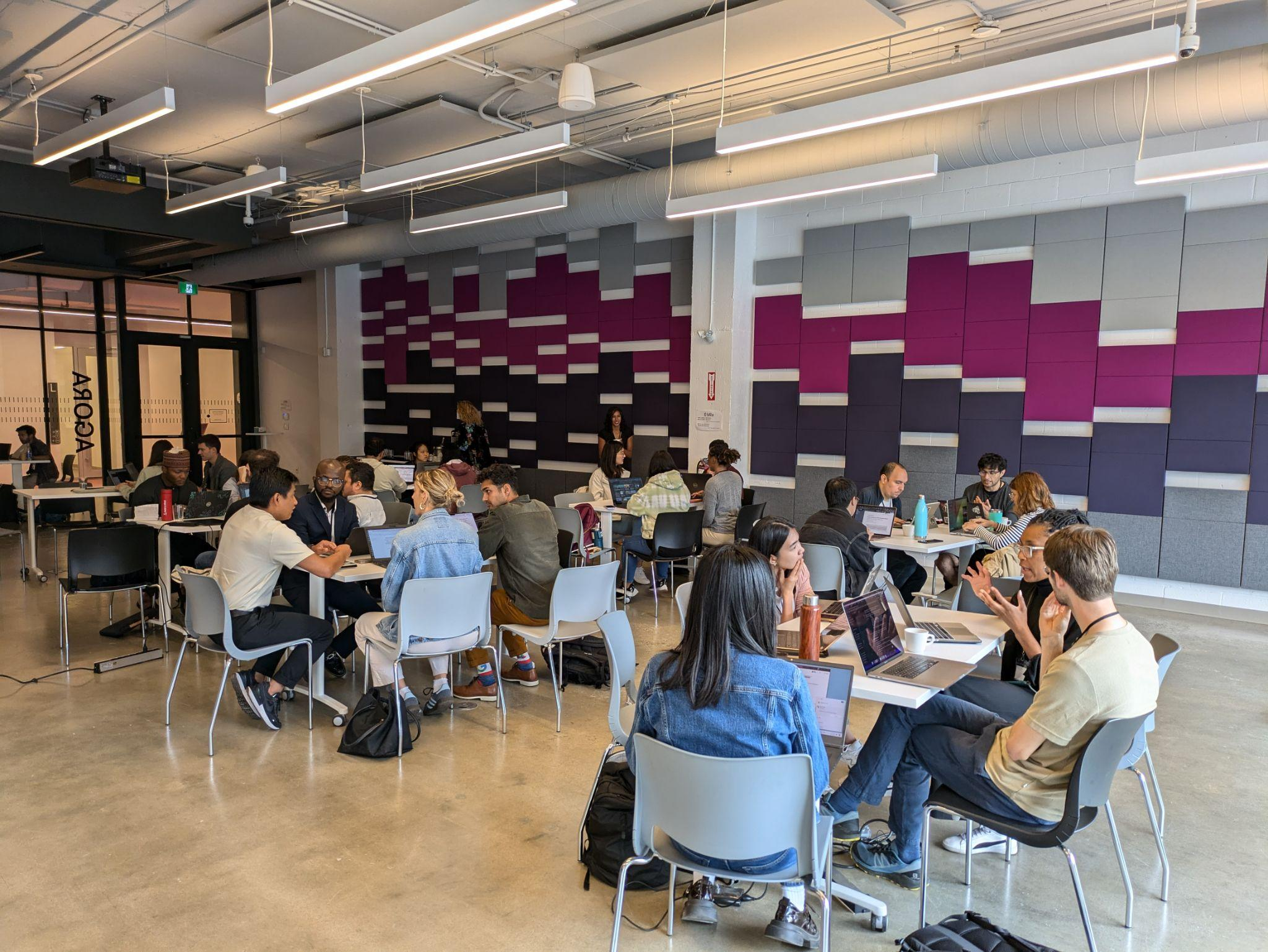
Participants formed ten interdisciplinary teams based on project proposals that they developed individually. Even before they arrived in Montreal, teams scoped out key aspects of the project together, such as the potential impact on climate change of the project and the stakeholders who may be affected by its implementation. They also identified the datasets necessary for their projects and how they would prepare those datasets to use during their project. With all of this prep work done, each team was able to hit the ground running when they arrived in Montreal. Outside of training sessions and social events, participants spent the majority of the week working with their teams. Supported by expert mentors, each team put significant effort into developing their projects and preparing for the final deliverable: the pitch event.
Pitch Event
After a week of hard work, each team was prepared to present their project to the rest of the cohort and a panel of expert judges. We heard from one team about increasing the lifespan and efficiency of photovoltaic modules by forecasting energy production, detecting degradation patterns, and predicting module failures. Another team presented their own flood prediction models in Kenya, which would provide an early warning system and potentially help Kenya to build a national flood management system. One team even demonstrated their climate vulnerability index through a live interactive demo! At the bottom of this page you will find a description of all the projects the participants worked on. And the work does not end with the Summer School: multiple teams submitted and had their projects accepted to the Tackling Climate Change with Machine Learning workshop at NeurIPS and may continue to develop these projects further.
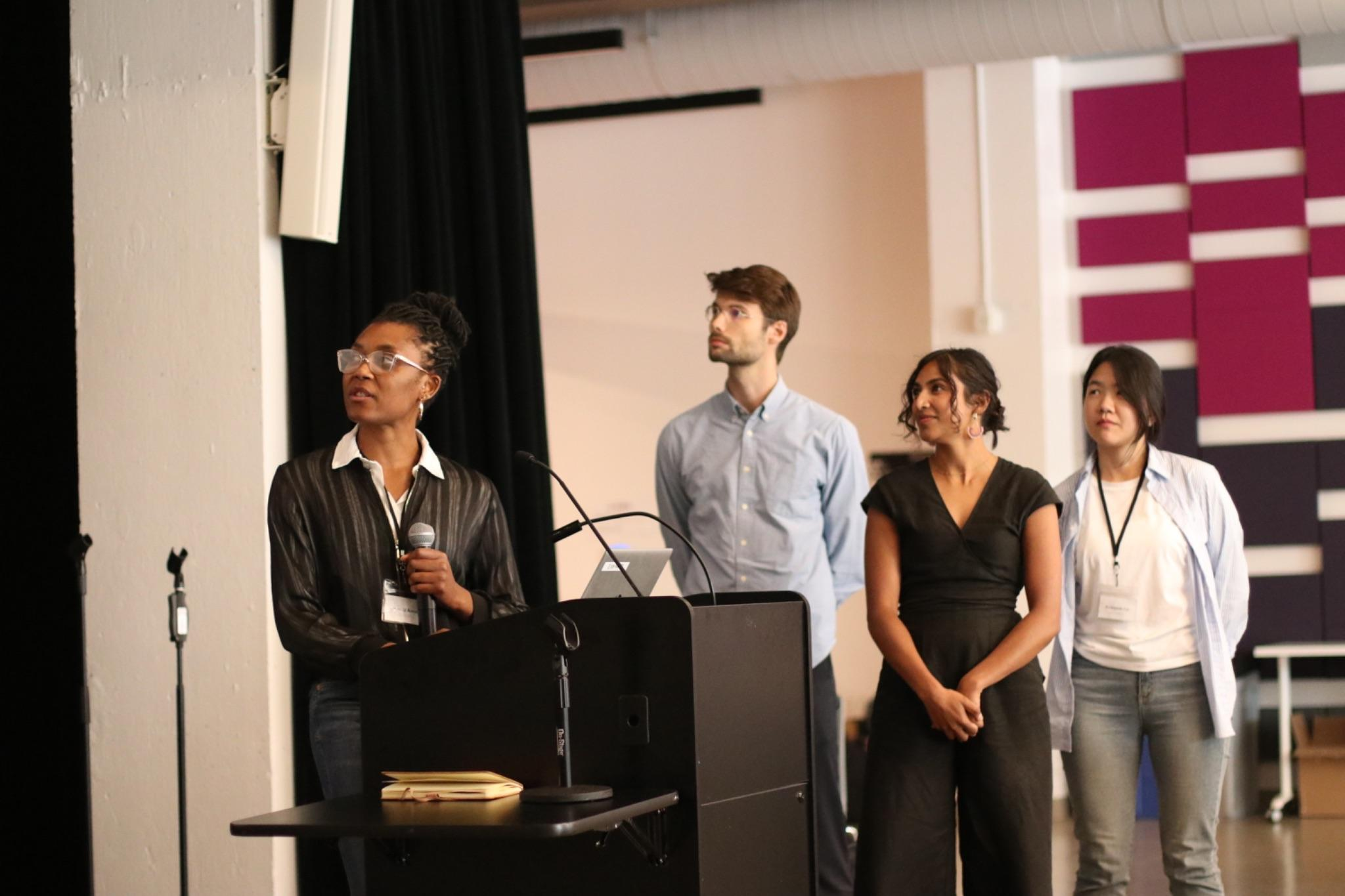
Goodbye, For Now
This year’s In-Person Summer School was truly an enriching experience for everyone involved. The participants’ drive for developing solutions for climate related problems using machine learning was inspiring, and their connections and work will extend far into the future. Before parting ways, many of us gathered for one final dinner, where we enjoyed great conversations and celebrated everyone’s terrific work. It was a great way to wrap up the program and say goodbye - at least, for now.
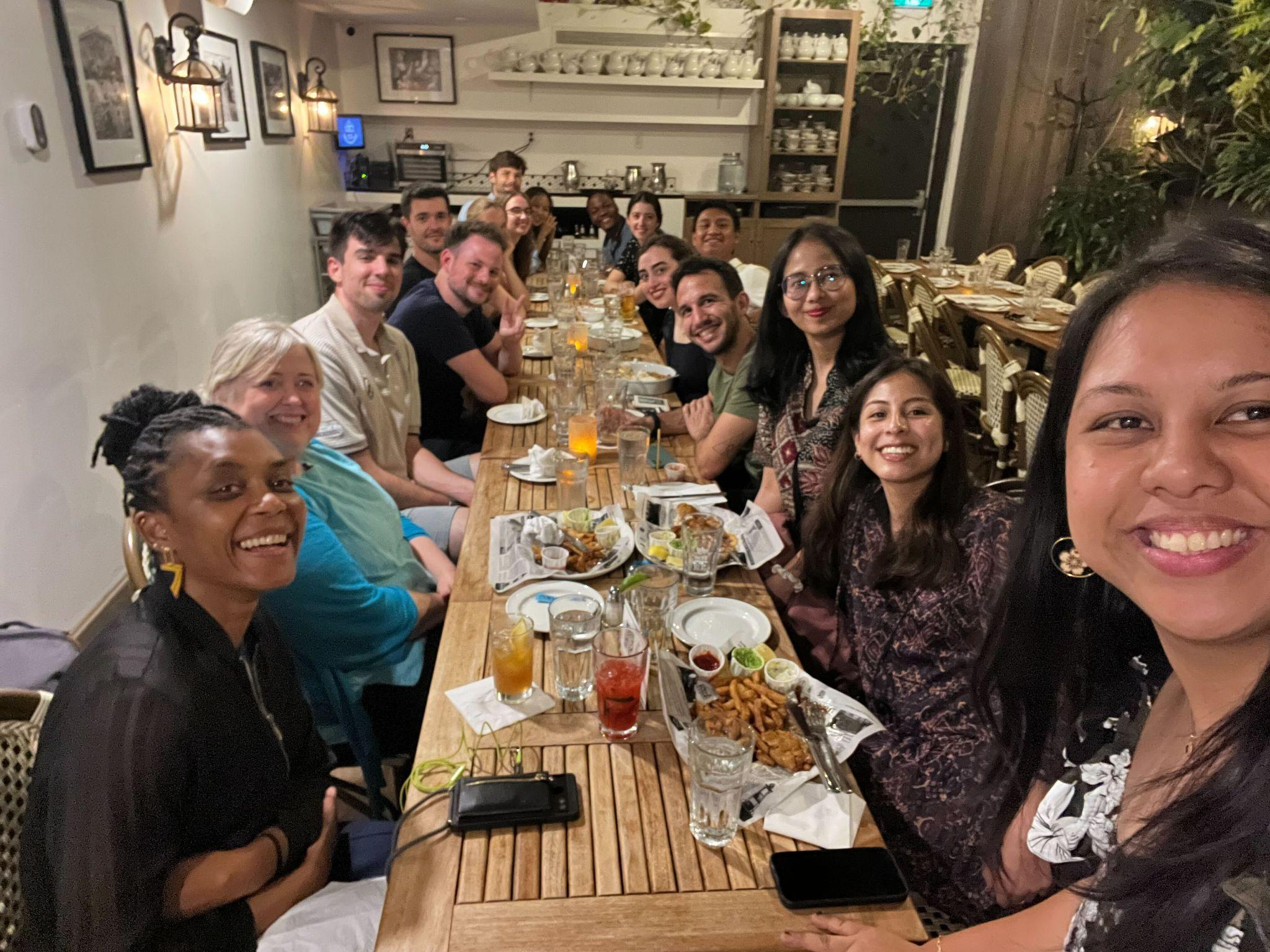
Interested in participating in the next iteration of the In-Person Summer School? Sign up here to be notified when our next application call is released.
Project Descriptions:
Lifespan and Efficiency of Solar Photovoltaic Modules
Giulia Lombardi, Andrés F. Pérez, Alvar Herrera, Christian Tran
The sustainability of future energy relies on the performance of photovoltaic (PV) modules, often hindered by degradation. This research seeks to improve the lifespan and efficiency of PV modules by using artificial intelligence (AI) and machine learning (ML) to develop predictive models based on historical performance data. These models will identify degradation sources affecting energy production, enabling optimized maintenance schedules, cost reductions, and minimized environmental impact. Specifically, we aim to use existing datasets to forecast energy production, detect degradation patterns, and predict module failures, supporting proactive maintenance and enhancing the reliability of solar power.
Downscaling For Improved Precipitation Forecasts In Data-Scarce Regions
Miriam Simm, Rajesh Kandel, Rogelio Barrios, Akram Zaytar
Climate change is expected to increase the frequency and intensity of extreme precipitation events, posing significant threats globally. Accurate and reliable precipitation forecasts are crucial for mitigating these impacts. While Numerical Weather Prediction (NWP) models provide global forecasts, their coarse resolution limits the accurate prediction of precipitation. Machine Learning (ML) based downscaling methods have emerged as a promising way forward,
though their application in data-scarce regions remains underexplored.
This study aims to develop an ML-based model in order to improve precipitation forecasts for the Karnali river basin in western Nepal, a data-scarce region vulnerable to extreme weather events. By training on high-resolution NWP simulations and fine-tuning with local observations, we aim to enhance forecast accuracy and reliability. The approach’s generalizability will be evaluated on other data-limited regions, with the goal of creating a flexible downscaling toolbox for broader application.
Climate Vulnerability Compass: Leveraging Machine Learning for Climate Vulnerability Assessment and Actionable Recommendations
Flávio Nakasato Cação, Isabel Drummond, Donna Marie Mlyneck
This paper proposes a framework that utilizes climate vulnerability indices, large language models (LLMs), and information retrieval (IR) systems to generate actionable recommendations for mitigating and adapting to potential environmental disasters. By integrating quantitative data from pre-existing indices with qualitative insights from policy documents, this project aims to equip public managers, journalists, and civil society with practical tools for understanding and addressing climate risks in specific regions. The ethical implications and pathways to impact of this approach are also discussed. Our prototype is available here: https://github.com/nakasato/climate-vulnerability-compass
Flood Prediction in Kenya
Alim Karimi, Hammed A Akande, Valerie Brosnan, Nicole Mong’are, Asbina Baral
We aim to build a flood prediction model which can be used by Kenyan authorities to deploy an early warning system. We aim to build two models. The first model will be predicting flooding events within a 900 square kilometer region. The second model will predict flooding events on a
much coarser 900 square meter region. Given that Kenya does not have a national flood management system, we hope that these technical advances can aid in building a national flood management system in Kenya and also in other countries which lack the infrastructure.
Advancing Climate Resilience in the Global South Through Enhanced Satellite Imagery
Zhining Gu, Muhammad Zawish, Eloise Lopez, Briah Davis
While High Resolution (HR) Remote Sensing (RS) imagery plays an important role in advancing climate resilience in various applications, such as damage estimation, crop yield estimation, and farm boundary delineation, its acquisition is prohibitively expensive. Fortunately, the cutting-edge Super Resolution (SR) techniques provide low-cost solutions to generate HR images from Low Resolution (LR) data. In the field of RS, due to the availability of ground-truth data (HR images) and sufficient financial support, SR models are frequently applied to the global north, but limited to developing countries across the global south. As a result, the improvement of climate resilience (i.e., disaster response, agriculture monitoring and management) in the global south is largely restricted.
To solve this issue, we leverage Open Data Program launched by MAXAR, one of the largest RS imagery producers, to curate HR and LR image pairs for the global south as benchmark datasets. The evaluation on various state-of-the-art SR models will be conducted. With the trained models, we further apply them to various downstream tasks. Finally, this project promises to improve the accessibility to HR satellite imagery for developing countries in the global south, assisting in various climate-related applications, such as disaster response, farm boundary delineation, crop type classification, and so on.
Understanding Crop Dynamics in Kenya
Hannah Wang, Nabila Putri Salsabila, Nirdesh Sharma
Kenya’s agriculture is vital, supporting much of the economy and employing a large part of the population. However, it faces serious challenges from climate change and food insecurity. This project aims to improve the health of Kenya’s soil by predicting soil organic carbon (SOC), a key indicator of soil fertility, using advanced data techniques like satellite imagery and machine learning. We start by identifying areas where crops are most likely grown using global land cover data. Then, we develop a machine learning model that not only maps crop types across the country but also includes a feature that measures the certainty of its predictions. This means the model can flag areas where it is less confident, helping us focus on getting the most accurate results. By combining these detailed crop maps with other data, such as soil and climate information, the project has already improved the accuracy of SOC predictions. Ultimately, this will help farmers adopt better farming practices, guide policy decisions, and contribute to global efforts against climate change, all while ensuring the benefits reach everyone, especially marginalized communities.
Monitoring the evolution of glacier surface area in the Peruvian Andes
Codrut-Andrei Diaconu, Gabriela Yaulli
Our project aims to develop a fully automatic pipeline to monitor the evolution of glacier surface area in the Peruvian Andes over a specific period of time. The goal is to provide annually updated area estimates for each glacier in the region, together with a tool that can be used by researchers who build glacier inventories to simplify their work. As a major part of our project, we plan to create a dashboard to visually represent changes in glaciers over time. We also aim to incorporate existing research findings that project how glaciers will change by the end of the century. This will provide a comprehensive view of both recent changes and potential future scenarios. The dashboard can be used as printed information for tourists and can also be made accessible on the web. This web version can provide continuously updated results and use animations to enhance the presentation.
From insight to action: Empowering residential users to lower their carbon emissions
Shivani Chotalia, Kyungmin Lee, Victor Hutse, Soazig Kaam
Residential buildings account for 17-20% of global greenhouse gas (GHG) emissions, posing a significant challenge in the effort to transition all buildings to net zero. While solutions such as heat pumps, building retrofits, and load shifting can significantly reduce emissions, homeowners are often unaware of their energy usage and the potential for cost reduction. Current methods for assessing individual building energy use typically rely on invasive approaches such as walk-throughs, surveys, and in-situ measurements, which can pose challenges related to data access and technology literacy, particularly for users. Supervised machine learning methods have the potential to provide actionable insights for individual energy-end users, encouraging them to reduce both GHG emissions and energy costs. We propose a novel framework that utilizes baseline, intervention, and optimization models to predict emissions and cost estimates for individual energy-end users. This paper presents a novel application of an optimization model for energy bills through machine learning methods: (1) classification of time series data for electricity and gas usage baselines, (2) prediction of GHG emission reductions, and (3) prediction of energy cost reductions.